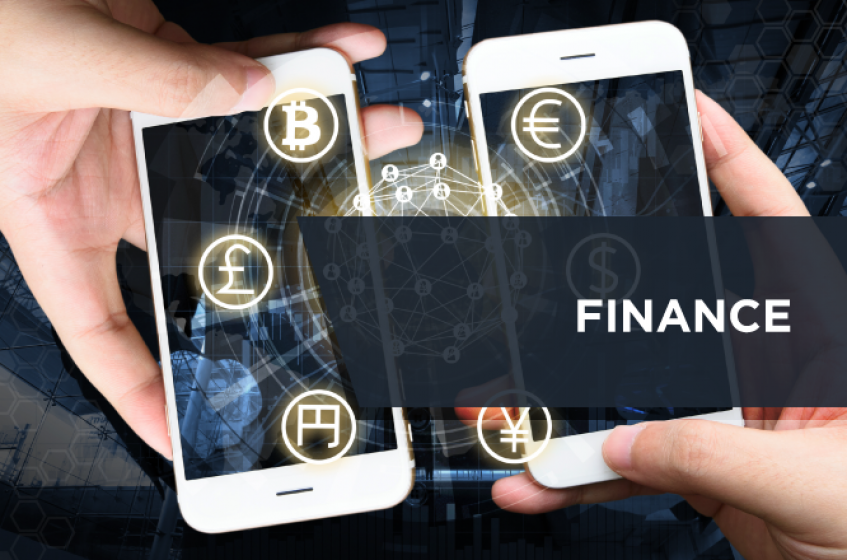
Maxing Out Entropy: A Conditioning Approach
Dr. Yan Liu
Associate Professor of Finance
Purdue University
Entropy and entropy-like measures of pricing kernel dispersion emerge as useful tools in asset pricing research. We develop a systematic approach to bounding entropy by incorporating conditioning information. Our bounds feature a fixed-point solution to a dynamic asset allocation problem. Similar to how the Sharpe ratio provides variance bound in the L2-space, our solution is interpretable as generalized “Sharpe ratios” in the entropy space. Importantly, as opposed to relying on physical moments alone, our bounds strike a balance in exploiting physical return predictability and hedging risk neutral higher order moments. Applying our approach to recently proposed return predictors, we document enhanced entropy restrictions that more than double the benchmark equity risk premium. We highlight the implications of our results in diagnosing leading macro-finance models.