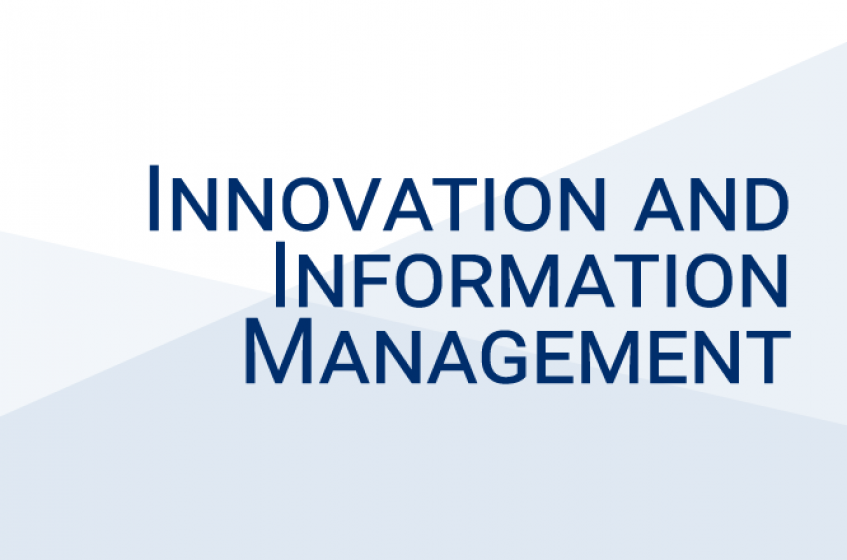
“Semiparametric and Robust Factor Analysis Speaker” by Dr. Weichen Wang
Dr. Weichen Wang
PhD in Operations Research and Financial Engineering (Statistics)
Princeton University
High-dimensional factor analysis is an important tool for modeling panel data. In this talk, we extend the approximate factor model in two meaningful ways.
Firstly, We propose a flexible semiparametric factor model, which decomposes the factor loading matrix into the component that can be explained by subject-specific covariates and the orthogonal residual component. We then introduce the Projected Principal Component Analysis (Projected-PCA), which employs principal component analysis to the projected data matrix onto the given linear space spanned by the covariates. When it applies to the semiparametric factor model, the projection removes noise components. We show that the unobserved latent factors can be more accurately estimated than the conventional PCA if the projection is genuine. Specifically, the factors can be estimated accurately even when the sample size is finite, which is particularly appealing in the high-dimension-low-sample-size situation. The proposed method is illustrated by both simulated data and the financial market equity data.
Secondly, we consider the heavy-tailed factor model, where the factor and residual return distributions may have heavy tails. In this case, we care to estimate the large-scale covariance matrix based on the approximate factor model. We propose a general Principal Orthogonal complEment Thresholding (POET) framework, where a set of high-level sufficient conditions for the procedure to achieve optimal rates of convergence under different matrix norms is established. Such a framework allows us to recover existing results for sub-Gaussian factor model in a more transparent way. As a new theoretical contribution, for the first time, such a framework allows us to exploit conditional sparsity covariance structure for the heavy-tailed data. The technical tools developed in this paper are of general interest to high-dimensional principal component analysis. Thorough numerical results are also provided to back up the developed theory.