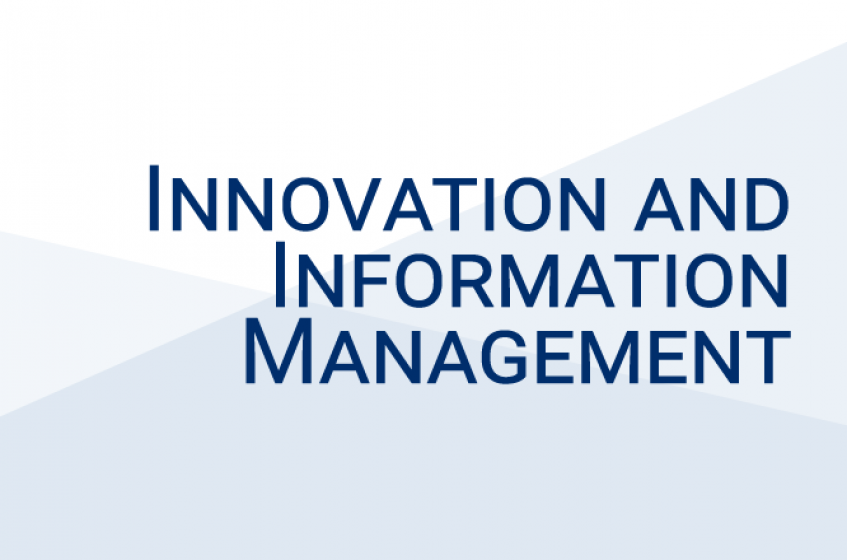
“Shepherding the Herd” by Dr. Jussi Keppo
Dr. Jussi Keppo
Associate Professor
Department of Analytics & Operations
National University of Singapore
This article analyzes multiple experts who forecast an underlying dynamic state based on a stream of public and private signals. Each expert minimizes a convex combination of her forecasting error and deviation from the other experts' forecasts. As a result, the experts exhibit herding behavior – a bias that has been well-recognized in the economics and psychology literature. Our first contribution derives and analyzes the experts' optimal forecast in a dynamic setting and under different levels of herding. This extends the Kalman filter and smoothing to applications where the underlying dynamics can be non-linear and herding is an important part of the process. Our second contribution is a welfare analysis. In particular, we show that less frequent reporting of public information and shocks to public information can be detrimental and have long lasting effects if the experts exhibit strong levels of herding. Our third contribution shows how the model can be estimated from data. The estimation methodology is illustrated on synthetic and real-world inflation forecasting data. The analysis shows that herding among inflation forecasters is substantial and can be mitigated by anonymous reporting. Finally, we introduce a simple compensation scheme that uses the estimated model to minimize the negative effects of herding.