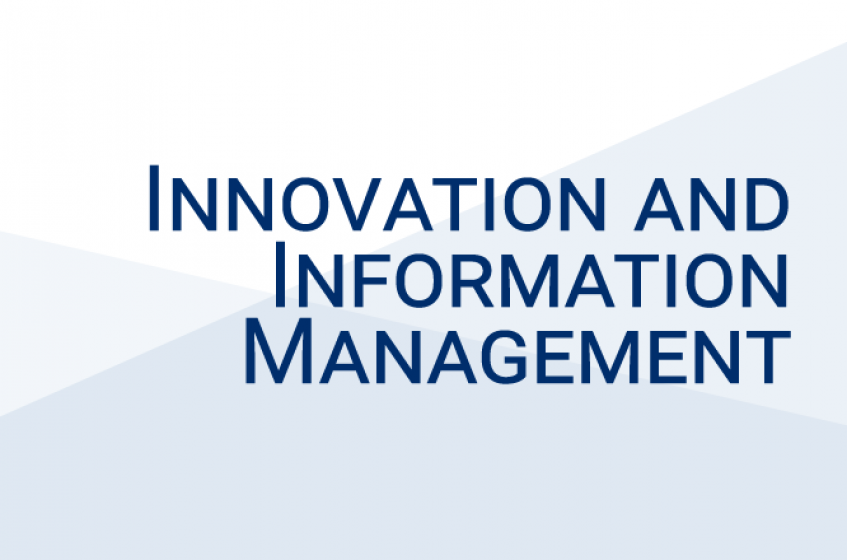
“Towards Data-driven Chronic Disease Management: A Diabetes Case Study” by Dr. Bin Liu
Research Scientist
Center for Computational Health
IBM Thomas J. Watson Research Center
Abstract: The recent abundance of medical record data has provided an unprecedented opportunity to apply artificial intelligence (AI) methods to create clinical decision support tools. In particular, accurate risk prediction could enable healthcare professionals to appropriately adapt preventive and personalized interventions, improving care quality and reducing cost. Given the large potential impact, it is valuable to develop risk prediction models that are accurate (predictions are statistically valid) and interpretable (easy to understand the rationale behind the predictions).
In this talk, Dr. Bin Liu will introduce data-driven AI methods to predict adverse health events for chronic disease management with a focus on diabetes. Diabetes is a chronic disease that affects nearly half a billion people around the globe, and often results in multiple complications, including kidney failure, stroke, and heart attack. Meanwhile, about 75% of all diabetes care expenditures ($327 billion in 2017) are spent on the treatment of complications. Risk prediction of diabetes complications is critical for improved diabetes management. He will first present a data-driven method to predict the risk of complications after the initial diabetes diagnosis using medical record data. They proposed TREFLES, a novel multi-task learning (MTL) model, to simultaneously model multiple complications where each task corresponds to the risk modeling of one complication. TREFLES provides a powerful tool for not only improving predictive performance, but also for recovering clinically meaningful insights about relationships among different risks and risk factors. As a further step to understand the risk factors, he will then present a private-shared MTL to identify outcome-specific risk factors attributable to each individual complication.