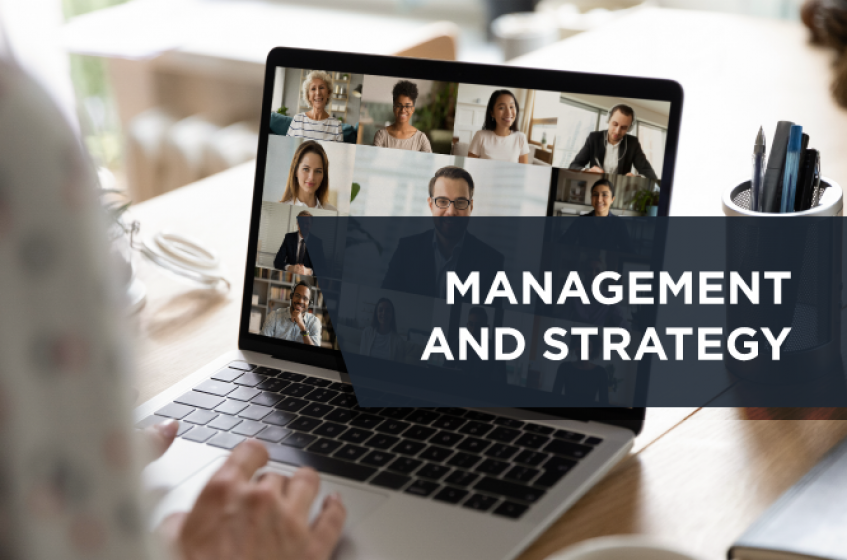
Using Machine Learning to Generate Novel Insights in the Organisational Sciences: Applications to Innovation and Entrepreneurship
Dr. Krishna Savani
Associate Professor of Leadership, Management, and Organization
Nanyang Business School
Nanyang Technological University
When management researchers want to explain important outcomes (e.g., employees’ job performance, countries’ innovation, and investors’ support for new ventures), they typically focus on one or a few antecedents suggested by prominent theoretical frameworks in the field. However, researchers might or might not identify the best explanation through this process. Machine learning methods are ideally suited for identifying the best explanation—they learn to predict the outcome of interest using all the available information and can identify the most important antecedents. I argue that machine learning can thus complement the traditional hypothetico-deductive reasoning that dominates the field. In the first project that I will describe today, I trained deep learning models to predict countries’ innovation scores and support for crowdfunding from residents’ responses to 680 attitudes, values, and beliefs included in the World Values Survey. The model could do so with 90% accuracy. Follow-up analyses revealed national pride as antecedents of both country-level innovation and support for crowdfunding. Follow-up experiments verified that increasing people’s national pride increased their creativity, a key driver of innovation, and increased their support for crowdfunding initiatives. Overall, this research highlights that machine learning methods can generate novel theoretical insights in the organizational sciences.