We introduce a learning model in which the decision maker does not know how recommendations are generated, called the contraction rule. We present behavioral postulates that characterize it. The contraction rule can be uniquely identified and reveals how the decision maker interprets and how much she trusts the recommendation. In a dynamic stationary setting, we show that the contraction rule is not dominated by completely following recommendations and is incompatible with a property called compliance with balanced recommendations. Following this negative result, we demonstrate that the contraction rule may generate and reinforce recency bias and disagreement.
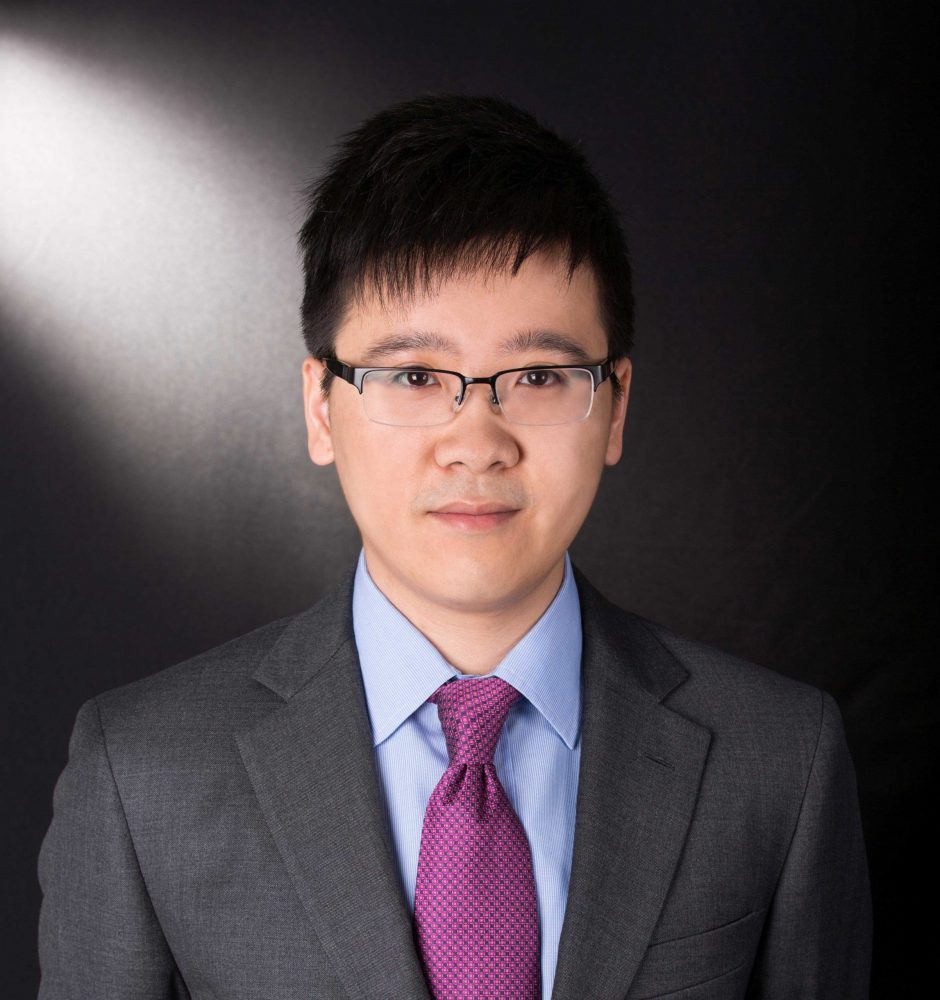
2857 8564
KK 924
We build on AGM belief revision (Alchourrón et al. (1985)) and propose a class of updating rules called pragmatic rules. Pragmatic updating applies to multiple priors and requires that the agent's posteriors be the subset of her priors under which the realized event occurs with probability 1, if such priors exist. We construct a propositional language based on qualitative probability and demonstrate the strong relation between belief updating rules and belief revision rules in this language. We show that an updating rule is consistent with AGM belief revision if and only if it is pragmatic. While maximum likelihood updating is pragmatic in general, full-Bayesian updating is not. We characterize maximum likelihood updating within the AGM framework, and show that full-Bayesian updating can be obtained by dropping one of AGM's postulates.