If a financial asset’s price movement impacts a firm’s product demand, the firm can respond to the impact by adjusting its operational decisions. For example, in the automotive industry, automakers decrease the selling prices of fuel-inefficient cars when the oil price rises. Meanwhile, the firm can implement a risk-hedging strategy using the financial asset jointly with its operational decisions. Motivated by this, we develop and solve a general risk-management model integrating risk hedging into a price-setting newsvendor. The optimal hedging strategy is calculated analytically, which leads to an explicit objective function for optimizing price and “virtual production quantity” (VPQ). (The latter determines the service level—that is, the demand-fulfillment probability.) We find that hedging generally reduces the optimal price when the firm sets the target mean return as its production-only maximum expected profit. With the same condition on the target mean return, hedging also reduces the optimal VPQ when the asset price trend positively impacts product demand; meanwhile, it may increase the VPQ by a small margin when the impact is negative. We construct the return-risk efficient frontier that characterizes the optimal return-risk trade-off. Our numerical study using data from a prominent automotive manufacturer shows that the markdowns in price and reduction in VPQ are small under our model and that the hedging strategy substantially reduces risk without materially reducing operational profit.
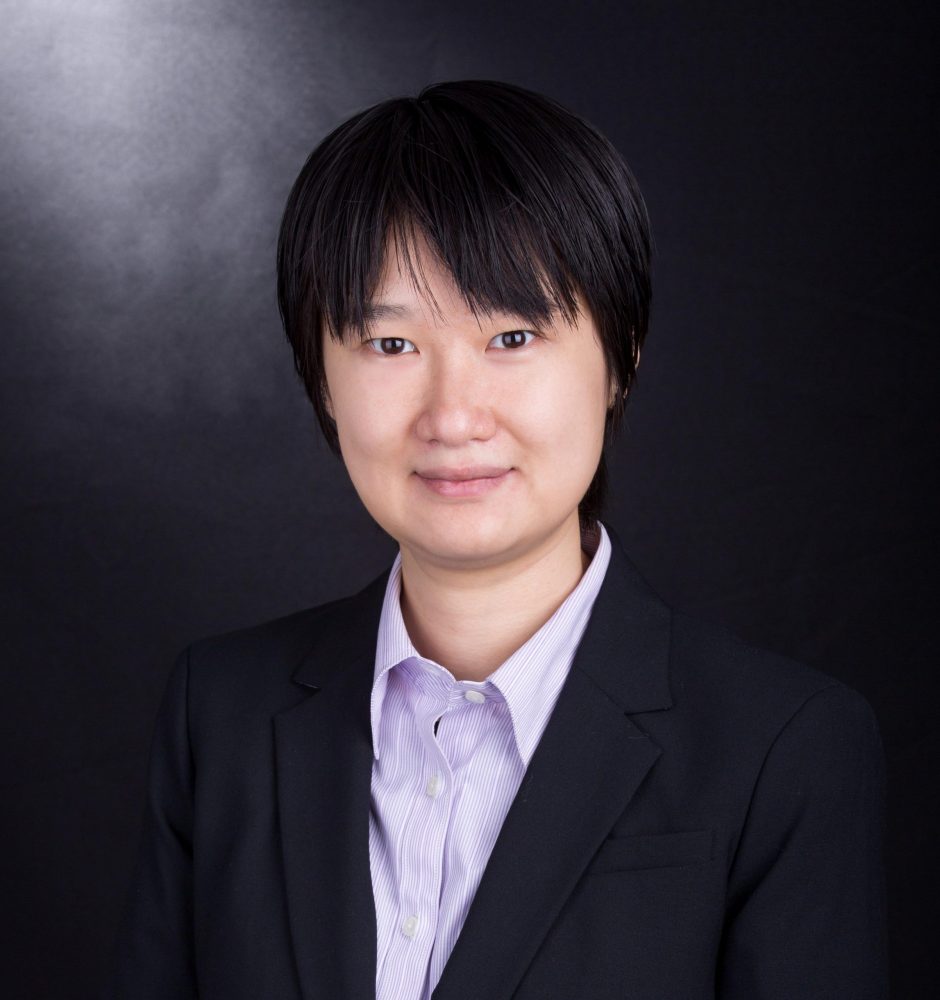
3917 1535
KK 806
- PhD in Operations Research, Columbia University
- Master in Financial Engineering, Cornell University
- Bachelor in Quantitative Finance, National University of Singapore
Liao Wang obtained her Ph.D. degree in Operations Research in Department of Industrial Engineering and Operations Research at Columbia University in 2017. Dr. Wang holds a BSc (Hons) in Quantitative Finance from National University of Singapore and MEng in Financial Engineering from Cornell University.
IIMT3636 – Decision and Risk Analysis I
MSBA7017 – Financial Engineering
- OM- finance/financial Engineering interface
- Risk analytics
- OM-innovation interface
- Wang, L., Yao, J., Zhang, X. W., “How Does Risk Hedging Impact Operations? Insights from a Price-setting Newsvendor Model”, Management Science, accepted for publication, 2023.
- Wang, L., Yao, D.D., “Production Planning with Risk Hedging Under a Conditional Value at Risk Objective”, Operations Research, 2023, Vol. 71(4), p1055-1072.
- Wang, L., “Mean-Variance Hedging for Production Planning with Multiple Products”, Production and Operations Management, 2021, Vol. 30(10), p3497-3522.
- Wang, L., Yao, D.D., “Risk Hedging for Production Planning”, Production and Operations Management, 2021, Vol. 30(6), p1825-1837.
- Wang, L., Yao, D.D., “Production with Risk Hedging: Optimal Policy and Efficient Frontier”, Operations Research, 2017, Vol. 65(4), p1095-1113.
- Wang, L., Wissel, J., “Mean-Variance Hedging with Oil Futures”, Finance and Stochastics, 2013, Vol. 17(4), p641-683.
MSBA Admission Committee
A central problem in planning production capacity is how to effectively manage demand risk. We develop a model that integrates capacity planning and risk hedging decisions under a popular risk measure, conditional value at risk (CVaR). The CVaR objective generalizes the usual risk-neutral objective (such as the expected payoff) and allows for explicit modeling of the degree of aversion to downside risk (associated with low demand). The starting point of our model is to incorporate the impact on demand from a financial asset (including for instance, a tradable market index as a proxy for the general economy). This way, in addition to the capacity decision at the beginning of the planning horizon, there is also a dynamic hedging strategy throughout the horizon, and the latter plays the role of both mitigating demand risk and supplementing the payoff. The hedging strategy is restricted to partial information and constrained with a cap on loss (pathwise). To find the optimal hedging strategy, we construct and solve a dual problem to derive the optimal terminal wealth from hedging; the real-time hedging strategy is then mapped out via the martingale representation theorem. With the hedging strategy optimized, we show that optimizing the production quantity is a concave maximization problem. With both production and hedging (jointly) optimized, we provide a complete characterization of the efficient frontier and quantify the improvement over the production-only model. Furthermore, via sensitivity and asymptotic analyses, we spell out the impacts of the loss cap and the risk aversion level, along with other qualitative insights.