Although financial fraud detection research has made impressive progress because of advanced machine learning algorithms, constructing features (or attributes) that can effectively signal fraudulent behaviors remains a challenge. In recent years, a new type of fraud has emerged on peer-to-peer (P2P) lending platforms, where individuals can borrow money from others without a financial intermediary. In these markets, the information asymmetry problem is seriously elevated. Inspired by the fraud triangle theory and its extensions, and using the design science research methodology, we construct five categories of behavioral features directly from P2P lending transaction data, in addition to the baseline features regarding borrowers and loan requests. These behavioral features are intended to capture the fraud capability, integrity, and opportunity of fraudsters based on their loan requests and payment histories, connected peers, bidding process characteristics, and activity sequences. Using datasets from real users on two large P2P lending platforms in China, our evaluation results show that combining these additional features with the baseline features significantly enhances detection performance. This design science research contributes novel knowledge to the financial fraud detection literature and practice.
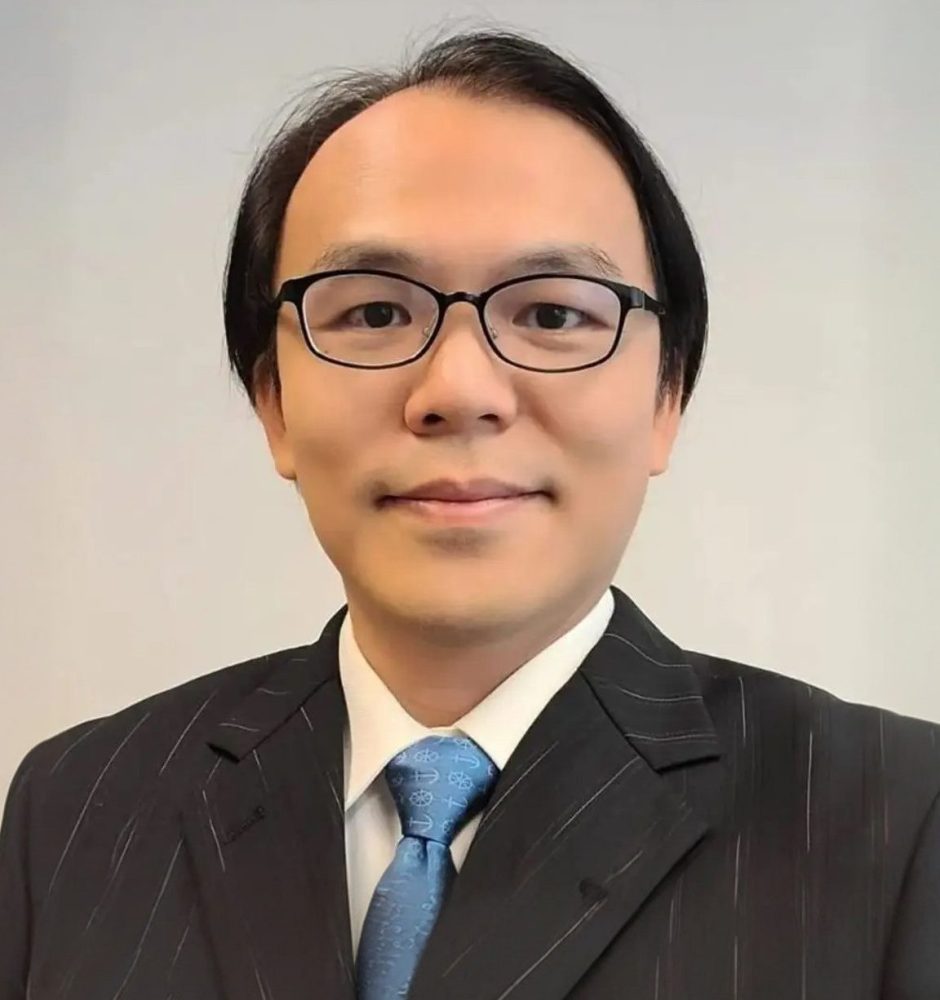
3917 1014
KK 1324
Emotional expressions have been widely used in online news. Existing research on the perception of online news has primarily focused on the effect of contextual cues on readers’ reasoning and deliberation behavior; the role of discrete emotions such as anger and sadness, however, has been overlooked. This paper addresses this research gap by investigating the influence of angry and sad expressions in online news on readers’ perception of the news. Drawing on the emotions as social information (EASI) theory and the appraisal-tendency framework (ATF), we find that expressions of anger in online news decrease its believability. However, sad expressions do not trigger the same effect. A further test reveals that the effect of angry expressions can be explained by the readers’ perception of the author’s cognitive effort: readers perceive that expressions of anger in the headlines denote a lack of cognitive effort of the author in writing the news, which subsequently lowers the believability of the news. We also show that news believability has downstream implications and can impact various social media behaviors including reading, liking, commenting, and sharing. This research extends current knowledge of the cognitive appraisals and interpersonal effects of discrete emotions (i.e., anger, sadness) on online news. The results also offer practical implications for social media platforms, news aggregators, and regulators that need to manage digital content and control the spread of fake news.
Online auction markets host a large number of transactions every day. The transaction data in auction markets are useful for understanding the buyers and sellers in the market. Previous research has shown that sellers with different levels of reputation, as shown by the ratings and comments left in feedback systems, enjoy different levels of price premiums for their transactions. Feedback scores and feedback texts have been shown to correlate with buyers’ level of trust in a seller and the price premium that buyers are willing to pay (Ba and Pavlou 2002; Pavlou and Dimoka 2006). However, existing models do not consider the time-order effect, which means that feedback posted more recently may be considered more important than feedback posted less recently. This paper addresses this shortcoming by (1) testing the existence of the time-order effect, and (2) proposing a Bayesian updating model to represent buyers’ perceived reputation considering the time-order effect and assessing how well it can explain the variation in buyers’ trust and price premiums. In order to validate the time-order effect and evaluate the proposed model, we conducted a user experiment and collected real-life transaction data from the eBay online auction market. Our results confirm the existence of the time-order effect and the proposed model explains the variation in price premiums better than the benchmark models. The contribution of this research is threefold. First, we verify the time-order effect in the feedback mechanism on price premiums in online markets. Second, we propose a model that provides better explanatory power for price premiums in online auction markets than existing models by incorporating the time-order effect. Third, we provide further evidence for trust building via textual feedback in online auction markets. The study advances the understanding of the feedback mechanism in online auction markets.
Dr Michael Chau’s study uses the machine learning and rule-based classification components of artificial intelligence to find people online who are in emotional distress. Using these techniques may help health professionals locate struggling individuals faster, getting help to them before it’s too late.
Many people face problems of emotional distress. Early detection of high-risk individuals is the key to prevent suicidal behavior. There is increasing evidence that the Internet and social media provide clues of people’s emotional distress. In particular, some people leave messages showing emotional distress or even suicide notes on the Internet. Identifying emotionally distressed people and examining their posts on the Internet are important steps for health and social work professionals to provide assistance, but the process is very time-consuming and ineffective if conducted manually using standard search engines. Following the design science approach, we present the design of a system called KAREN, which identifies individuals who blog about their emotional distress in the Chinese language, using a combination of machine learning classification and rule-based classification with rules obtained from experts. A controlled experiment and a user study were conducted to evaluate system performance in searching and analyzing blogs written by people who might be emotionally distressed. The results show that the proposed system achieved better classification performance than the benchmark methods and that professionals perceived the system to be more useful and effective for identifying bloggers with emotional distress than benchmark approaches.
The health and medical needs of an ageing population mean Hong Kong has to store up increasing amounts of fresh blood products. This year alone, the Hong Kong Red Cross Blood Transfusion Service (BTS) needs 3.4 per cent more units of whole blood, plasma and platelets than it collected last year, when demand increased by 4.4 per cent. Added to that growing demand is the fact that donations from first-time donors have fallen, in part because the revised school curriculum means Form 7 students are now spread out in universities and the workplace, rather than easily targeted in a school. So where can additional donors be found? To help find an answer, the BTS has been turning to Dr Michael Chau, Associate Professor in the Faculty of Business and Economics, whose research focuses on data mining and analysis.