On-demand service platforms are interested in having gig workers use self-set, nonbinding performance goals to improve efforts and performance. To examine the effects of such self-set goal mechanisms, we build a behavioral model, derive theoretical results and testable hypotheses, and conduct a field experiment using a large gig platform for food delivery. Our model analysis finds that individual workers’ optimal self-set goals may exhibit a spectrum of difficulty levels, ranging from trivial to impossible, depending on workers’ reference-dependent utility coefficients and self-control cost. Moreover, workers’ efforts are higher with properly set goals rather than no-goals. Consistently, our experimental data show significant treatment effects of self-goal setting, and a causal tree algorithm identifies subgroups who are mostly motivated by self-set goals. Furthermore, our study compares two common types of performance metrics for goal setting: the number of completed orders and total revenue. Our model suggests different cases of effort and performance improvement for the two goal types. The experimental data suggests that both goal types improve efforts equally but lead to different attainment rates. Specifically, the goal attainment rate is lower for the revenue-goal treatment than for the order-quantity-goal treatment. Further analysis reveals that this disparity is due to workers setting excessively high revenue goals. Our study demonstrates the efficacy and limitations of self-goal-setting mechanisms and yields two important managerial implications. First, the implementation of self-goal-setting mechanisms could improve gig workers’ efforts and performance. Second, encouraging order-quantity goals instead of revenue goals could help gig workers achieve higher attainment rates.
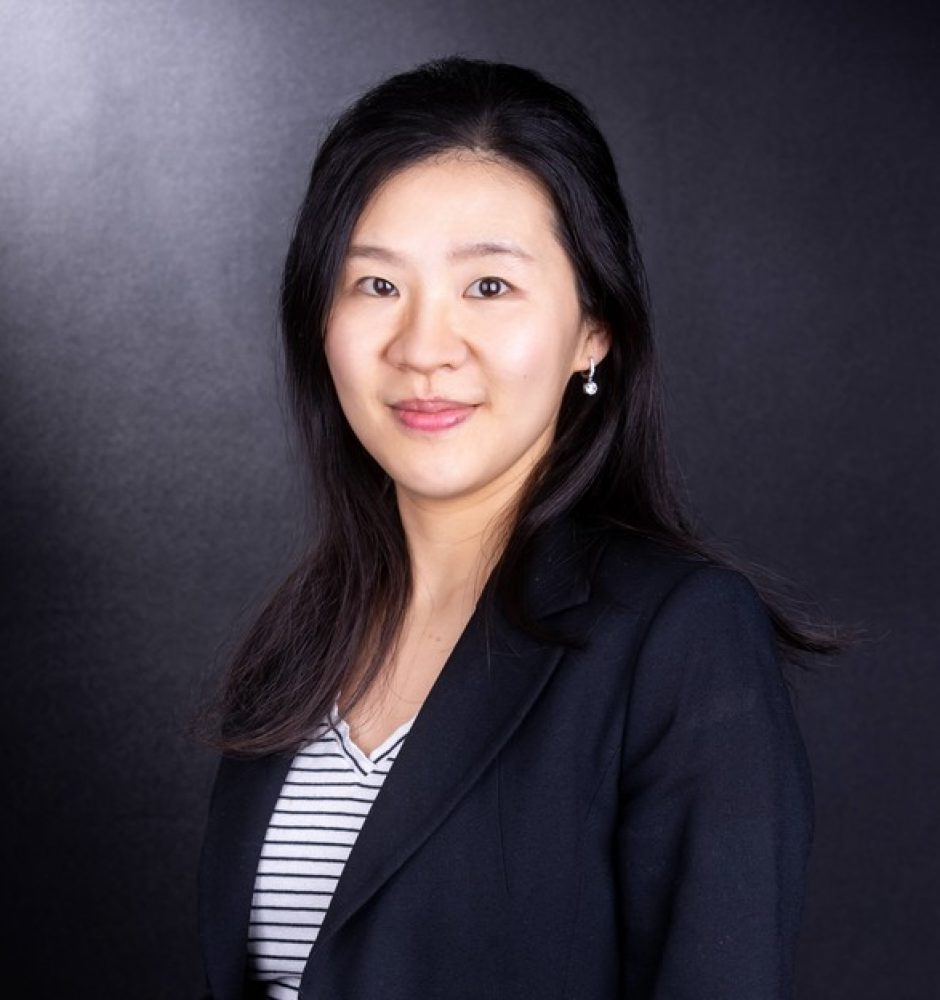
Problem definition: We study the use of nonmonetary incentives based on reciprocity to facilitate capacity sharing between two service providers that have limited and substitutable service capacity. Academic/practical relevance: We propose a parsimonious game theory framework, in which two firms dynamically choose whether to accept each other’s customers without the capability to perfectly monitor each other’s capacity utilization state. Methodology: We solve the continuous-time imperfect-monitoring game by focusing on a class of public strategy, in which firms’ real-time capacity-sharing decision depends on an intuitive and easy-to-implement accounting device, namely the current net number of transferred customers. We refer to such an equilibrium as a trading-favors equilibrium. We characterize the condition in which capacity sharing takes place in such an equilibrium. Results: We find that some degree of efficiency loss (as compared with a central planner’s solution) is necessary to induce reciprocity. The efficiency loss is small when the two firms have similar traffic intensity even if they are different in service-capacity scale, whereas the efficiency loss can be considerably large when the two firms have significantly different traffic intensities. The trading-favors mechanism, surprisingly, can outperform the perfect-monitoring benchmark when the two firms exhibit high asymmetry in terms of service-capacity scale or traffic intensity because the smaller firm tends to deviate from collaboration. Managerial implications: Firms should consider engaging in nonmonetary reciprocal capacity sharing if regulations, transaction costs, or other market and operational frictions make it difficult to use a capacity-sharing contract based on monetary payments. The trading-favors collaboration can improve the firms’ payoff close to the centralized upper bound when the firms have similar traffic intensities. However, when their traffic intensities are highly different, firms are better off with a monetary-payment contract to induce more capacity sharing and are worse off investing in increasing their visibility to each other’s real-time available capacity, namely investing in perfect monitoring.