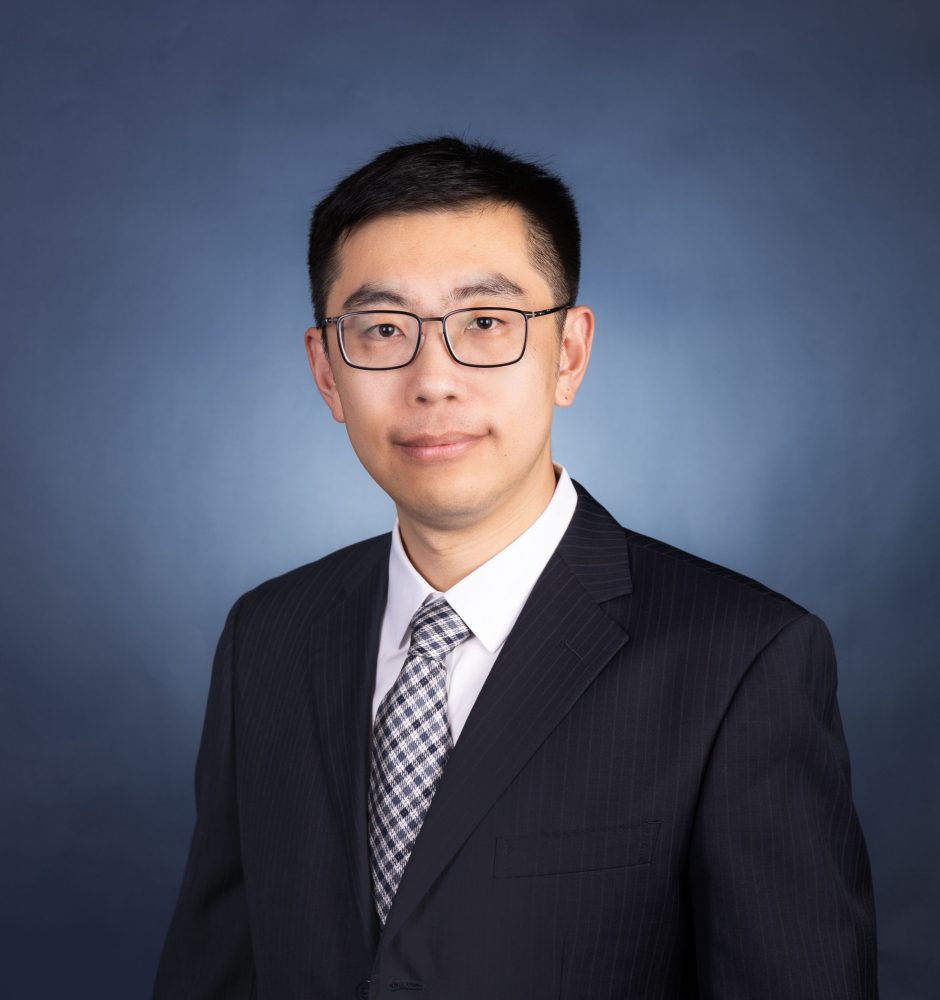
Prof. Xinghao Qiao
Innovation and Information Management
Associate Professor
3910 3109
KK 1340
Academic & Professional Qualification
- PhD in Business Statistics, Marshall School of Business, University of Southern California
- MS in Statistics, University of Chicago
- BS in Mathematics and Physics, Tsinghua University
Biography
Xinghao Qiao is an Associate Professor in the area of Innovation and Information Management at the HKU Business School. Prior to HKU, he was an Associate Professor in the Department of Statistics at London School of Economics.
Teaching
- Probability and Statistics for Business (HKU)
- Machine Learning and Data Mining (LSE)
- Artificial Intelligence (LSE)
- Regression and Generalized Linear Models (LSE)
- Applied Business Statistics (USC)
Research Interest
- Functional data analysis
- Complex time series analysis
- High-dimensional statistics/econometrics
- Machine learning in finance
Selected Publications
- Guo, S., Li, D., Qiao, X. and Wang, Y. (2025). From sparse to dense functional data in high dimensions: Revisiting phase transitions from a non-asymptotic perspective. Journal of Machine Learning Research, 26(15), 1-40.
- Chang, J., Fang, Q., Qiao, X., and Yao, Q. (2024). On the Modeling and Prediction of High-Dimensional Functional Time Series. Journal of the American Statistical Association, in press.
- Liu, Y., Qiao, X., Wang, L. and Pei, Y. (2024). Deep functional factor models: Forecasting high-dimensional functional time series via Bayesian nonparametric factorization, the 41th International Conference on Machine Learning, PMLR, 235, 31709-31727.
- Fang, Q., Guo, S. and Qiao, X. (2024). Adaptive functional thresholding for sparse covariance function estimation in high dimensions. Journal of the American Statistical Association,119, 1473-1485.
- Chang, J., Chen, C., Qiao, X. and Yao, Q. (2024). An autocovariance-based learning framework for high-dimensional functional time series. Journal of Econometrics, 239, 105385.
- Liu, Y., Qiao, X., Wang, L. and Lam, J. (2023). EEGNN: Edge enhanced graph neural networks with a Bayesian nonparametric graph model, the 26th International Conference on Artificial Intelligence and Statistics, PMLR, 206, 2132-2146.
- Guo, S. and Qiao, X. (2023). On consistency and sparsity for high-dimensional functional time series with application to autoregressions. Bernoulli, 29, 451-472.
- Liu, Y., Qiao, X. and Lam, J. (2022). CATVI: Conditional and adaptively truncated variational inference for hierarchical Bayesian nonparametric models, the 25th International Conference on Artificial Intelligence and Statistics, PMLR, 151, 3647-3662.
- Fang, Q., Guo, S. and Qiao, X. (2022). Finite sample theory for high-dimensional functional time series with applications. Electronic Journal of Statistics, 16, 527-591.
- Chen, C., Guo, S. and Qiao, X. (2022). Functional linear regression: dependence and error contamination. Journal of Business & Economic Statistics, 40, 444-457.
- Lian, H., Qiao, X. and Zhang, W. (2021). Homogeneity pursuit in single index models-based panel data analysis. Journal of Business & Economic Statistics, 39, 386-401.
- Qiao, X., Qian, C., James, G. and Guo, S. (2020). Doubly functional graphical models in high dimensions, Biometrika, 107, 415-431.
- Qiao, X., Guo, S. and James, G. (2019). Functional graphical models. Journal of the American Statistical Association, 114, 211-222.
- Radchenko, P., Qiao, X. and James, G. (2015). Index models for sparsely sampled functional data. Journal of the American Statistical Association, 110, 824-836.