Differentially private multiple testing procedures can protect the information of individuals used in hypothesis tests while guaranteeing a small fraction of false discoveries. In this paper, we propose a differentially private adaptive FDR control method that can control the classic FDR metric exactly at a user-specified level α with a privacy guarantee, which is a non-trivial improvement compared to the differentially private Benjamini-Hochberg method proposed in Dwork et al. (2021). Our analysis is based on two key insights: 1) a novel p-value transformation that preserves both privacy and the mirror conservative property, and 2) a mirror peeling algorithm that allows the construction of the filtration and application of the optimal stopping technique. Numerical studies demonstrate that the proposed DP-AdaPT performs better compared to the existing differentially private FDR control methods. Compared to the non-private AdaPT, it incurs a small accuracy loss but significantly reduces the computation cost.
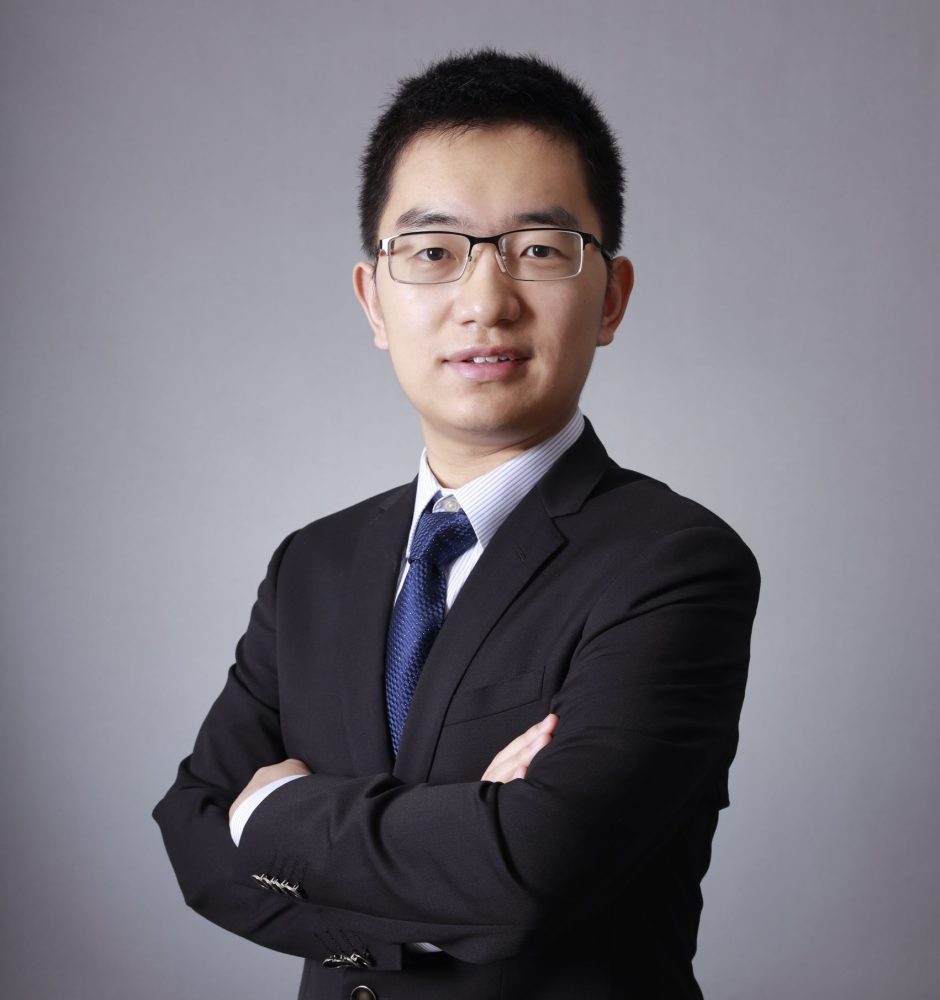
Prof. Zhanrui CAI
Innovation and Information Management
Assistant professor
3910 3104
KK 1336
Academic & Professional Qualification
- Ph.D. in Statistics (The Pennsylvania State University), 2021;
- Bachelor in Statistics (Renmin University of China), 2016.
Biography
Zhanrui Cai is an assistant professor in the area of Innovation and Information Management at the HKU Business School. Previously, he was an assistant professor in the Department of Statistics, Iowa State University.
Research Interest
- High dimensional inference
- Distribution-free inference
- Conformal inference
- Causal discovery
- Differential privacy
Selected Publications
- Awan, J., and Cai, Z. (2023) “One Step to Efficient Synthetic Data”, Statistica Sinica, forthcoming.
- Xia, X., and Cai, Z. (2023) “Adaptive False Discovery Rate Control with Privacy Guarantee”, Journal of Machine Learning Research, 24(252): 1-35.
- Cai, Z., Lei, J., Roeder, K. (2023) “Asymptotic distribution-free independence test for high dimension data”, Journal of the American Statistical Association.
- Cai, Z., Lei, J., Roeder, K. (2022) “Model-free prediction test with application to genomics data”, Proceedings of the National Academy of Sciences.
- Du, J., Cai, Z., Roeder, K. (2022) Robust probabilistic modeling for single-cell multimodal mosaic integration and imputation via scVAEIT, Proceedings of the National Academy of Sciences
- Cai, Z., Li, C., Wen, J., Yang, S. (2022) Asset splitting algorithm for ultrahigh dimensional portfolio selection and its theoretical property, Journal of Econometrics.
- Cai, Z., Zhang, Y., Li, R. (2022) “A distribution-free conditional independence test with application to causal discovery”, Journal of Machine Learning Research.
- Cai, Z., Xi, D., Zhu, X., Li, R. (2022) Causal discoveries for high dimensional mixed data, Statistics in Medicine.
- Tong, Z., Cai, Z., Yang, S., Li, R. (2022) Model-free conditional feature screening with FDR control, Journal of the American Statistical Association.
- Zhu, X., Cai, Z., Ma, Y. (2021) Network functional autoregression model, Journal of the American Statistical Association.
- Cai, Z., Li, R., Zhu, L. (2020) “Online sufficient dimension reduction through sliced inverse regression”, Journal of Machine Learning Research.
Recent Publications
1Jul
1 Jul 2023
Journal of Machine Learning Research