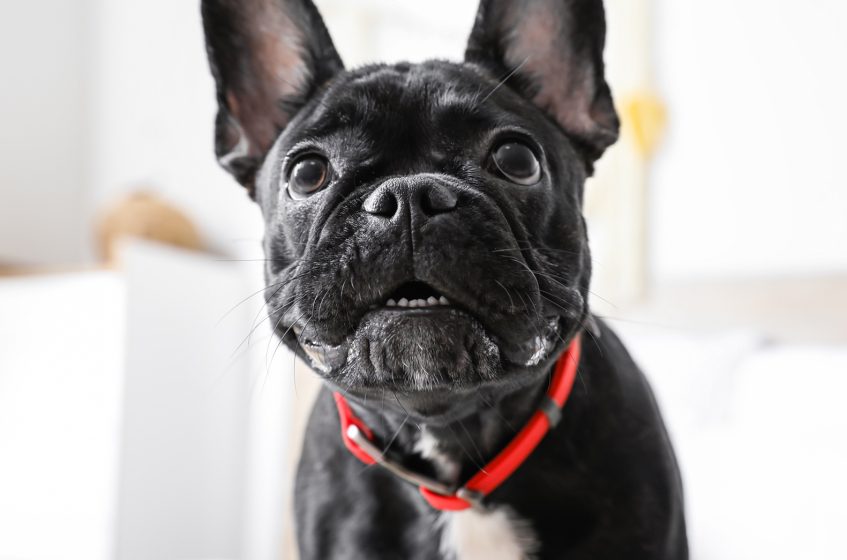
The Dark Side of Algorithms: From Big Data-Driven Price Discrimination to Tacit Collusion
The authors have found that pressuring businesses to implement a completely fair pricing system may actually backfire and lead to tacit collusion where consumers will ultimately suffer.
In today’s wave of big data, companies are building more complex advanced algorithms to categorise consumers and set different price points. The goal is to help businesses identify loyal customers and discriminate against them by charging them more. This practice has alerted regulators across the world. They have either drafted or enacted laws to clamp down on price discrimination behaviours to promote price fairness. Still, this pursuit of price fairness consequently shines a light on an emerging hidden risk: companies could use pricing algorithms to form tacit collusion and maintain monopoly pricing in a competitive market (see Note 1). Even though many regulatory bodies remain highly concerned about algorithmic discrimination and algorithmic collusion, very few studies have been conducted with a focus on crucial links between the two. As such, the authors of this article have delved deep into this problem and put forward important policy suggestions. They present fresh ideas on ways to tackle algorithmic discrimination and algorithmic collusion.
Regulatory Dilemma: Caught Between a Rock and a Hard Place
In 2018, the United Kingdom’s Financial Conduct Authority issued a discussion paper which highlighted a common and widespread problem in the country’s financial services industry – the “loyalty penalty”. This is where loyal customers are unable to enjoy special prices when they renew their contracts (see Note 2). Even if the risk levels of long-standing customers have not increased, insurers still charged them higher premiums. This practice often puts loyal customers in an even more unfavourable situation (see Note 3). In 2021, a court in China’s Zhejiang province ruled in favour of a consumer of Trip.com who accused the travel services provider of price discrimination. The plaintiff, a “Diamond member” with Trip.com, took legal action after being charged more than double the hotel’s room rate. Similar practices of big data price discrimination have also been heavily criticised by Chinese consumers (see Note 4). In response, China’s Ministry of Culture and Tourism issued new regulations in 2020, forbidding online booking platforms from taking advantage of their loyal users. Over in the United Kingdom, the Financial Conduct Authority also introduced new rules. From 2022, insurers have been banned from quoting customers a higher price for renewing their home or motor insurance when compared to a new customer.
While both policy intentions and regulatory means are fundamentally alike, their respective impact on the economy could not be more different. For the travel booking industry, the same type of hotel is generally listed on multiple platforms. This means different travel platforms end up providing homogenous products and competition remains relatively fierce. For industries with a high degree of homogeneity, barring price discrimination can often promote consumer surplus and further suppress a potential price collusion between businesses. However, when it comes to the insurance market, companies often develop products with varying terms, scope and length to set themselves apart from competitors. When it comes to these highly differentiated markets, imposing a completely fair pricing system could backfire: companies could use this to their advantage and collectively raise prices set by algorithms. This will lead to tacit collusion and consumers will ultimately suffer. In fact, not long after the Financial Conduct Authority issued new restrictions, the premiums on home and motor insurance registered their biggest month-on-month increase in the United Kingdom in over eight years (see Note 5). Such a difference serves as a reminder to regulators that perfect price fairness and an entirely competitive market are contradictory – Prohibiting behaviours of price discrimination may induce problems and hinder healthy market competition. This shows that regulators should exercise caution when dealing with social problems arising from algorithmic pricing and prevent causing a butterfly effect.
Future Regulations: Targeting Both the Symptoms and Root Causes
As businesses create more comprehensive algorithms, regulating price discrimination and tacit collusion will also require more targeted methods. The authors’ research provides two feasible regulatory routes:
First of all, restricting – rather than banning – price discrimination is a more stable and realistic approach. This approach can impair the ability of companies to engage in price collusion by stimulating competition. It can also improve social welfare more so than having an unregulated environment or a complete ban on price discrimination. Secondly, rather than pursuing absolute price fairness, regulators should consider adopting randomised pricing interventions and turn to probability on whether or not to impose price fairness. This kind of randomised regulation can also promote a higher degree of price fairness whilst weakening the chances of businesses colluding together. In addition, this research has emphasised on the need for regulators to rely on more advanced technologies to audit pricing algorithms and increase algorithmic transparency. When there is a two-pronged approach directed at algorithm abuse that facilitates discriminatory and predatory pricing; when fairness and anti-monopoly have been taken into full account; only then can we ensure that the whole society can enjoy technology dividends brought about by companies who make advances in product development.
The authors of this article are Prof. Xiao LEI, Assistant Professor in Innovation and Information of HKU Business School; Prof. Pin GAO, Assistant Professor of CUHK (Shenzhen) School of Data Science, Mr. Zongsen YANG, Ph.D. student of CUHK (Shenzhen) School of Data. This article is based on their joint research paper “Regulatory Discriminatory Pricing in the Presence of Tacit Collusion”.
Note 1: https://www.justice.gov/opa/pr/justice-department-and-federal-trade-commission-file-statement-interest-hotel-room
Note 2: https://www.fca.org.uk/publication/discussion/dp18-09.pdf
Note 3: https://www.bbc.com/news/business-57270415
Note 4: https://technode.com/2021/07/15/regulators-target-price-discrimination
Note 5: https://www.raconteur.net/finance/fca-ban-price-walking-rebound-consumers
Professor Xiao LEI
Assistant Professor in Innovation and Information, HKU Business School
Professor Pin GAO
Assistant Professor, School of Data Science, CUHK (Shenzhen)
Mr. Zongsen YANG
Ph.D. student in Data Science, CUHK (Shenzhen)
This article was also published on September 5, 2024 on the Financial Times’ Chinese website