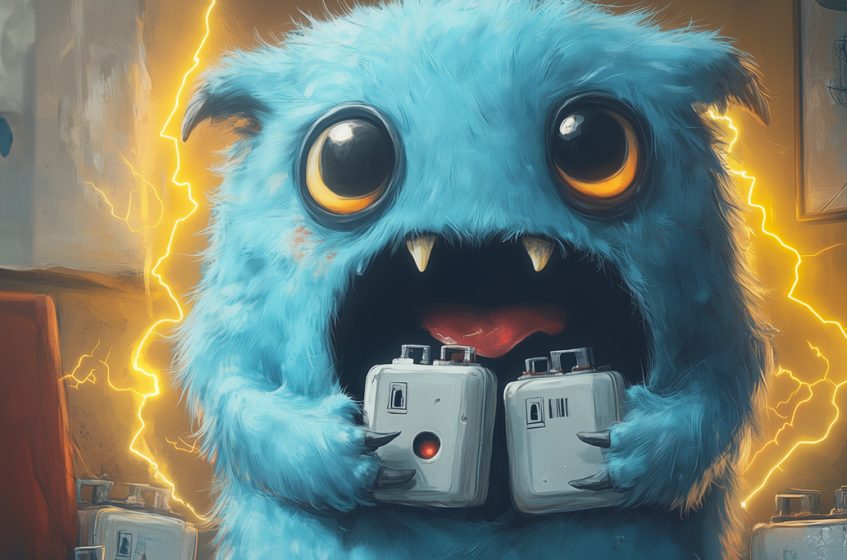
AI and Its Environmental Consequences: Can We Turn the Tide on Carbon Emissions?
AI衝擊環境 碳排放有何轉機
今時今日,人工智能(AI)技術突飛猛進,從智能助手到自動駕駛,從工業生產到醫療診斷,AI的應用已深入人類生活的方方面面。根據國際數據公司(International Data Corporation)預測,全球AI市場規模將從2022年的1324億美元增長到2027年的5124億美元。
在為AI帶來的創新便利歡呼之餘,社會各界是否意識到,這場科技革命正在悄然對地球環境造成巨大影響?事實上,AI發展過程中產生的碳排放問題,已經到了不容忽視的地步。
隱形殺手:AI訓練的碳足跡
要理解AI對環境的影響,必須揭開AI模型訓練的面紗。現代AI模型,尤其是大型語言模型的訓練過程,需要海量的數據支持和龐大的計算資源。根據馬薩諸塞大學阿默斯特分校的最新研究,訓練一個大型AI模型產生的碳排放量高達62.6萬磅,相當於5輛汽車從生產到報廢的全生命周期碳排放總和。
具體而言,GPT-3的訓練過程,大約產生552噸二氧化碳;至於規模更大的GPT-4,碳排放量估計超過1000噸。令人尤其擔憂的是,這個數字正在不斷攀升。在「大模型即王道」的行業共識下,各大科技公司爭相發展更大規模的模型,導致能源消耗呈指數級增長。到2030年,估計AI行業的碳排放量將佔全球碳排放的3.5%。
數據中心:AI時代的耗能巨獸
當前AI大模型的能源消耗令人咋舌。史丹福AI研究所數據顯示,GPT-3單次訓練耗電1287兆瓦時,相當於3000輛特斯拉電動車各行駛20萬英里的總耗電量,產生552噸二氧化碳排放。
從日常應用看,ChatGPT回應用戶1次,耗電量達2.96瓦時,差不多是標準谷歌搜索(0.3瓦時)的10倍;由AI驅動的谷歌搜索更需8.9瓦時。水資源消耗同樣驚人:GPT-3訓練期間,耗水近700噸,每20至50個問題就需500毫升水。2022年,Meta公司僅在數據中心冷卻上,就已耗水260多萬立方米。
人工智能大模型的高能源消耗主要源於兩大核心因素。首先,隨着AI技術的快速反覆運算,晶片需求量急升,直接推高了電力消耗。現代AI模型的訓練和推理過程使用大量計算資源,這些資源主要依賴高性能硬體,包括圖形處理單元(GPU)和專用集成電路(ASIC),運行時耗電極大,以應付複雜的計算。隨着AI模型規模不斷擴大,所需的計算能力也呈指數級增長,對高性能晶片的需求亦有增無已,令整體電力消耗量飆升。
再者,AI模型訓練過程需要強大的算力支持,這些運轉不息的數據中心產生高熱量,必須冷卻處理。中心作為AI計算的核心基礎設施,其能耗問題尤為明顯。伺服器和儲存設備在高負荷運行時會釋出巨大熱量,如果不及時散熱,設備的性能和壽命都會嚴重受損。因此,數據中心都配備高效的冷卻系統,以確保設備在適當溫度下操作。
數據中心營運成本結構中,電費佔總成本的60%,其中超過40%用於冷卻散熱系統;特別是在風冷數據中心,超過60%的電力用來散熱,真正用於計算的電力還不到40%。這種能源利用效率的失衡,導致全球數據中心的耗電量已達10年前的近10倍。傳統的風冷系統雖然成本較低,但效能亦較低,難以滿足現代數據中心的高效散熱所需。相比之下,液冷系統雖然初期投資較高,但散熱效率亦高,能夠大幅降低數據中心的能源消耗。
此外,中心的選址和設計也對能耗有重大影響。不少數據中心建在電力成本較低而氣候炎熱的地區,加重冷卻系統的負擔。為了提高能源利用效率,數據中心應優先選擇氣候涼爽、能源供應穩定的地區,並採用模組化設計,以便根據需求靈活調整計算資源的配置。
最後,AI模型的訓練和推理過程還涉及大量的數據傳輸和儲存,這也增加了能源消耗。隨着數據量持續增加,中心因需要更多儲存設備和更高頻寬才足以應付,而進一步推高能耗。由此可見,企業應積極採用數據壓縮和優化傳輸技術,減少不必要的程序,以便降低能耗。
面對AI技術帶來的環境挑戰,企業和政策制定者需要採取一系列減碳措施。一、企業應積極採用綠色能源和節能技術。通過投資太陽能、風能等可再生能源,減少對傳統化石燃料的依賴。二、企業應優化AI模型的訓練演算法,減少不必要的計算量,從源頭上降低能源消耗。三、企業應加強數據中心的管理和技術升級。採用液冷等高效冷卻技術,提升能源使用效率。通過智能調度和負載均衡,減少數據中心的閒置資源浪費。四、企業可通過虛擬化技術,整合計算資源,進一步降低能耗。
在政策層面,一、政府應制定嚴格的能效標準,推動AI技術的綠色發展。通過稅務優惠和資助,鼓勵企業採用節能技術和可再生能源。二、加強對數據中心的監管,制定能源效益評估標準,推動行業整體能效提升。三、政府和業界攜手推動公眾和企業的環保意識。通過碳排放交易、碳抵消等機制,減少AI技術對環境的負面影響。教育和宣傳是不可或缺的範疇,只有當AI發展與環境保護之間的關係廣為人知,才能形成社會共識,合力應對。
AI技術勢不可擋,但我們必須確保其發展不以犧牲環境為代價。通過科技創新、企業自律、政府引導和社會監督等多管齊下的方式,在享受AI便利的同時,亦可把環境影響降至最低。根據國際可再生能源機構的預測,若能採取積極措施,到2030年,AI行業的碳排放增長率可以控制在每年5%以內。
作為這個時代的見證者和參與者,我們每個人都應該關注AI發展帶來的環境問題,用實際行動支持綠色AI的發展。只有這樣,才能夠確保AI技術真正造福人類,而不會變成地球的另一個負擔。在追求科技進步的道路上,環境保護絕不應作為可有可無的點綴,反之應是必須嚴格遵守的底線。讓社會上下齊心協力,推動AI走向更加綠色、可持續的未來。
通過政策引導、科技創新和公眾參與,香港不難在2035年之前,實現AI行業的碳中和目標,為全球可持續發展作出貢獻。
本欄逢周三刊登
章逸飛博士
港大經管學院經濟學高級講師