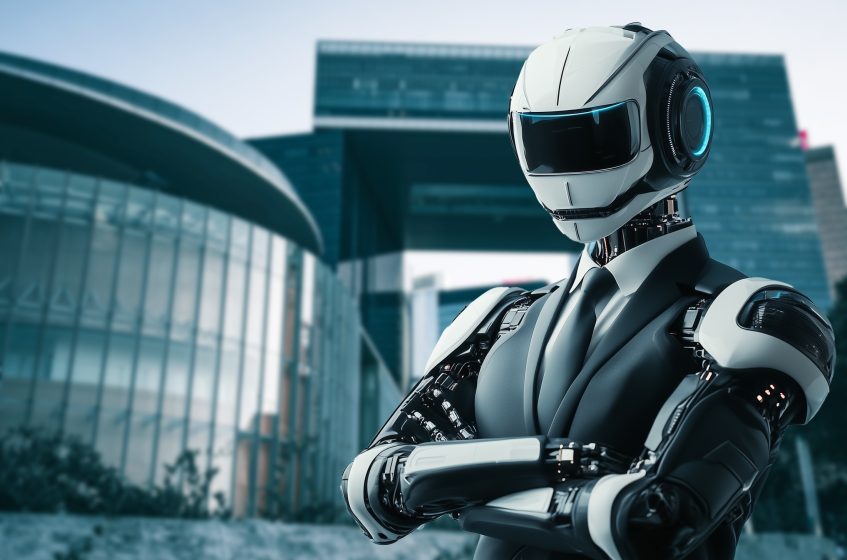
A New Chapter in Smart Governance: AI Empowering Innovation in Government Services
從人工視窗到智慧問答:大語言模型與RAG技術重塑政務服務
陳海亮、于淼:研究顯示RAG框架在處理政務查詢時,可支援更複雜的問題,提供準確度高的答案,而所需的時間、算力和成本亦遠低於增量預訓練和微調方法。
近年,類似於ChatGPT的大語言模型(Large Language Model,LLM)在全球迅速普及,展示出巨大的應用潛力。 通過海量數據的訓練,這些模型能夠生成連貫且語義合理的文本,並具有卓越的問答能力。 在政務服務領域,公眾一般通過政府官網、移動應用查詢政策法規、辦事流程等資訊,或者前往政務服務大廳求助。 傳統的政務服務主要依賴人工視窗服務和電話諮詢,服務效率和回應速度往往受到限制,尤其是在遇到複雜問題或需要長時間排隊等待時,公眾滿意度往往較低。 隨著技術的發展,特別是大語言模型的出現,政務服務逐漸邁進智慧化、自動化。 結合大語言模型的對話式諮詢服務更為高效,能顯著提升公眾體驗。
儘管大語言模型在處理常規問題時表現優異,但在涉及政策法規等專業領域時,仍面臨諸多挑戰。 LLM的訓練數據主要來源於互聯網的公開內容,缺乏深入專業知識,可能導致生成不準確或不符合實際情況的回答。 在某些特定場景下,模型可能會出現「幻覺」現象,即自信地提供錯誤資訊。 這種問題對普通用戶來說通常難以識別,因為他們未必具備相關領域的專業知識。 此外,政務政策經常更新變化,如果大語言模型未能及時獲取最新的政策資訊,其回答可能會與當前政策相衝突。 由此可見,為提高政務AI系統的可靠性,除了確保回答的準確性外,還需加強其可解釋性,以幫助使用者理解每個回答的依據,此舉亦有助提升系統的透明度和信任度。
RAG在政務服務中的應用特點
為了將大語言模型更好地應用於政務服務等場景,檢索增強生成(Retrieval-Augmented Generation,RAG)框架應運而生。 RAG技術通過兩個步驟優化答案生成:首先,檢索與用戶問題相關的文檔或文本片段; 其次,利用LLM基於這些檢索結果生成解答。 引入RAG解決了當前政務服務中的一些問題,並表現出一定的優勢【註1】。
首先,RAG通過集成外部知識庫,確保模型生成的答案基於最新且權威的政策法規文本,大幅提升回答的準確性。 其次,RAG支援更為複雜的查詢方式,能夠處理多層次和多維度的問題,不依賴單一的關鍵詞匹配。 通過結合檢索結果與生成內容,RAG不僅提高了信息的準確度,還增強了使用者對AI系統的信任度。 這樣,用戶可清晰地看到每個回答背後的依據,減少了幻覺現象帶來的困擾。 此外,RAG技術的應用大幅降低成本和算力消耗。 與增量預訓練(Incremental Pre-training)和微調(Fine-tuning)相比,RAG無需重新訓練大型模型,而是通過外部數據源的檢索與補充來提升生成品質。 因此,RAG框架在構建政務問答系統時所需的時間、算力和成本遠低於傳統的增量預訓練和微調方法。
基礎模型、微調模型與RAG框架:哪個最出色?
為了進一步評估RAG框架在政務法律領域的效果,筆者通過語義規則評分和事實一致性評分的對比分析,探討基礎模型、微調模型與RAG框架在生成內容品質及準確性方面的差異。 研究結果顯示,微調模型在語義評分方面表現最佳,全因其回答的語言風格和用詞更接近標準答案。 然而,微調模型存在一個顯明問題,其「幻覺」現象較基礎模型更為嚴重,生成的回答常包含許多與事實不符的內容,影響實際應用的可靠性。 與之相比,基礎模型生成的回答較為簡潔,資訊量相對較少。 在處理複雜問題時,能力有限,應對高精度任務時表現欠佳。 RAG框架則有效緩解幻覺現象,生成的回答中矛盾點明顯少於微調模型,並且提供更多準確的信息,事實一致性較強。 RAG框架在確保準確性和一致性的同時,能夠有效減少幻覺現象,特別適用於需要外部知識支援的複雜任務,如政務諮詢和分析。
在成本方面,經綜合分析性能與資源消耗后發現,微調模型的成本是RAG框架的五倍,而RAG框架的成本則與基礎模型相近。 RAG框架在確保生成質量的同時,亦能顯著降低成本,是效率與成本之間的理想選擇。 更關鍵的是,對於政府和企業而言,數據安全至關重要。 RAG框架可通過完全私有化部署的方式應用,無需將敏感內部數據上傳至第三方平臺,有效降低數據洩露的風險【註2】。
現時,RAG技術的政務問答助手,能夠有效應對傳統政務服務中的資訊滯後、檢索低效及人工干預等問題。 隨著技術持續進步,RAG系統預計在更廣泛的應用領域上進一步擴展其功能,提供更加個人化的政務服務,例如智慧內容推薦和數位人助手。 這不僅有助於實現低延遲的語音交互,還能依據使用者需求提供定製化的政務服務體驗,讓服務變得更個人化及精準。
本文作者為港大經管學院助理院長(碩士課程)、人工智慧研究所所長、創新及資訊管理學教授陳海亮以及港大經管學院博士生于淼。
註1:《智慧政務新篇章:AI助力政務服務創新》專題報告 https://fwik3jehaxr.feishu.cn/file/FFCjbsGLzoiHuOxKcTPcNqn1nMb
註2:《智慧政務新篇章:AI助力政務服務創新》專題報告 https://fwik3jehaxr.feishu.cn/file/FFCjbsGLzoiHuOxKcTPcNqn1nMb