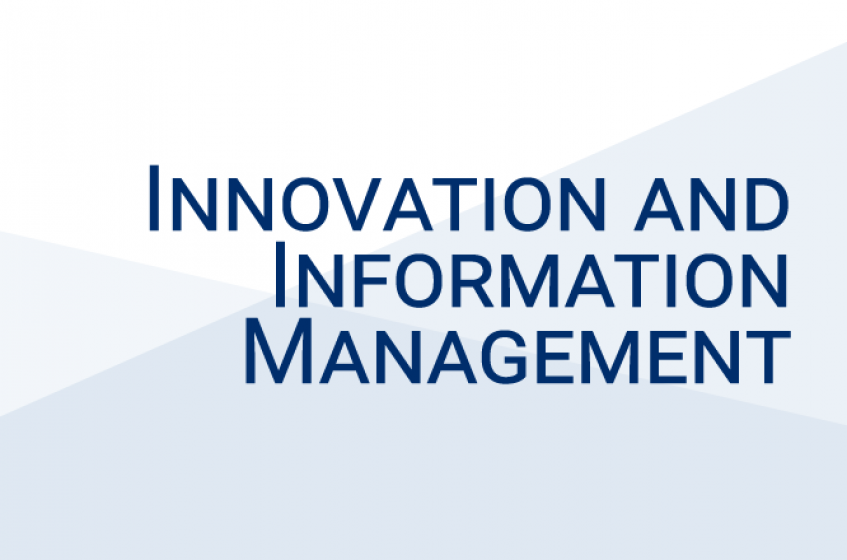
“Analysis of Consumer Technology Product Choice: An Integrated Approach to Combine Important Product Attributes and High-Level User Perceptions” by Prof. Paul Hu
David Eccles Chair Professor of Information Systems
University of Utah
Professor Hu plans to present two studies :
This study presents a novel approach, premised in hierarchical information integration, to analyze and explain consumer choice among alternative technology products or designs, according to estimates of user utilities.
The proposed approach explicates how people process technology product attributes (such as product features and usage context factors) to form high-level perceptions. These attributes and user perceptions then jointly determine consumer choice. Our approach is generic and can derive structural models for various consumer choice scenarios. Two choice-based conjoint experiments are designed and conducted to examine the predictive power of the proposed approach. The evaluation results show that the models derived from the proposed approach consistently better predict consumer choice than the benchmark models, measured by in-sample fit and the holdout prediction hit rate at both individual and aggregate levels. This study offers a viable, theory-based approach to model how consumers integrate information about essential technology product attributes to form high-level user perceptions, which together explain their choices. The findings also indicate a promising extension to hierarchical information integration by recognizing and modeling distinct influence paths of one product attribute to multiple high-level user perceptions and then to consumer technology choice.
Containing skyrocketing health care costs is imperative. Toward that end, prescriptive analytics that analyzes health care data to recommend optimal decisions is both relevant and crucial. We propose a novel prescriptive analytics method to improve the cost effectiveness in clinical decision making (CDM), a critical health care dimension that can greatly benefit from analytics. Effective prescriptive analytics for CDM has to address its probabilistic, cost-sensitive, and investment-related characteristics simultaneously. Unlike existing methods that often overlook the investment-related characteristic, the proposed method accounts for all of these characteristics. Specifically, our method considers two sets of costs associated with clinical decisions-before and after an investment-in combination with the probabilities of cost changes due to the investment. In contrast, prevalent methods only emphasize one set of costs, before an investment. Furthermore, the proposed method specifies both clinical and investment decisions, whereas existing methods ignore investment decisions.
Empirical evaluations with two real-world clinical datasets indicate that the proposed method consistently and significantly outperforms several prevalent methods from previous research, thereby demonstrating the value of addressing the investment-related characteristic in efforts to improve CDM.