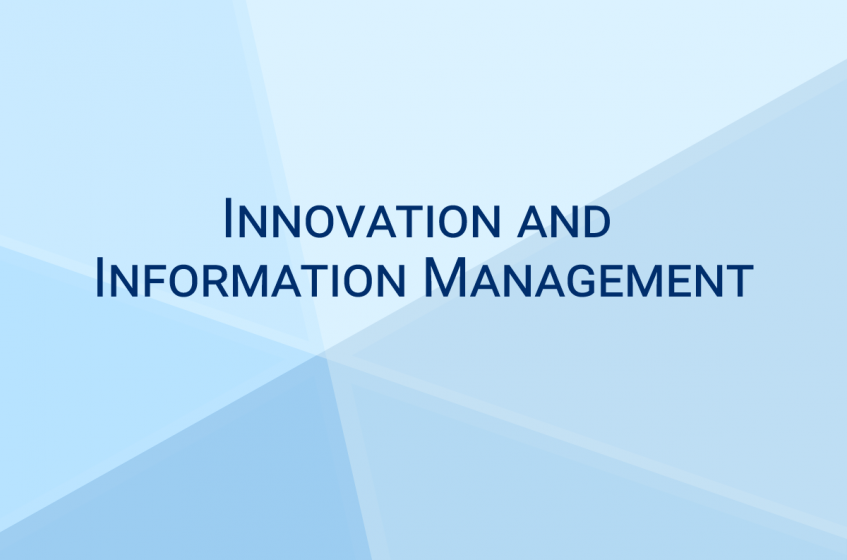
Coordinated Care: Capacity Allocation to Improve Itinerary Completion in Queueing Networks
Assistant Professor of Management
Krannert School of Management
Purdue University
Under the burgeoning paradigm of coordinated care, healthcare networks comprised of an integrated suite of specialist services are becoming more popular and face an increasingly diverse patient demand. This growing business model requires a sophisticated capacity allocation scheme for managing patients with different target deadlines for completing their itinerary of care in the network. In this work, we collaborate with the Mayo Clinic to develop a discrete‐time queueing network to optimize the capacity allocation to improve itinerary completion rates. We characterize the itinerary completion time with a doubly‐stochastic phase‐type distribution and leverage a mean‐field model to address computational intractability. We integrate the itinerary completion time model within a policy improvement framework to approximately solve a large‐scale stochastic optimization that maximizes the proportion of on‐time itinerary completions. We demonstrate, via a case study of the Mayo Clinic, that our solution approach can significantly improve on‐time completion, from 70% under the current capacity allocation to more than 95% using our approach. If time permits, I will also discuss some ongoing works on model inference and performance prediction in queueing networks and applications to COVID19 surge planning.