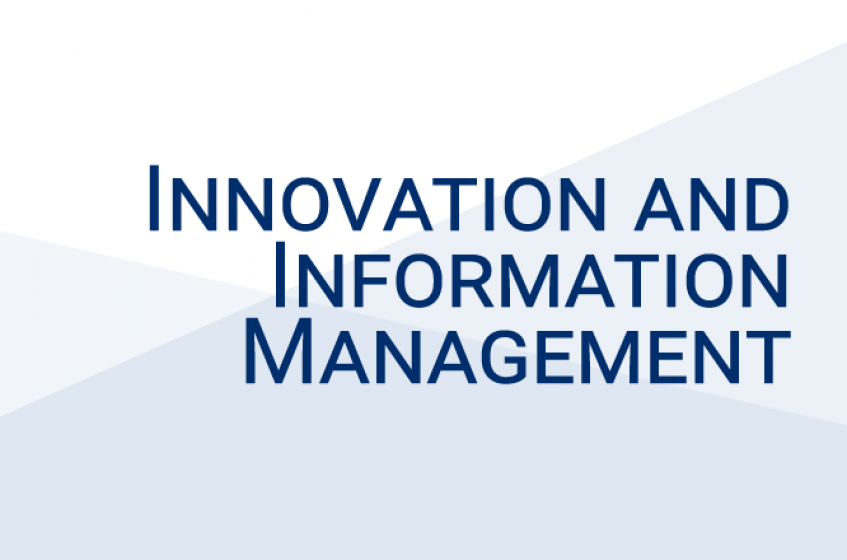
“Data-Driven Robust Decision-Making” by Dr. Zhi Chen
Research Associate in Management
Imperial College Business School
In the era of modern business analytics, data-driven robust optimization (DDRO) has emerged as a popular modeling paradigm to transform data into decisions. By constructing an ambiguity set of the potential data-generating distributions and subsequently hedging against all member distributions within this ambiguity set, DDRO effectively combats the ambiguity that real-life data sets are plagued with. Amongst others, successful applications of DDRO have been reported in operations management, machine learning, portfolio selection, energy systems, and medical decision-making.
In this talk, I propose two DDRO optimization models where the ambiguity set comprises all distributions in a Wasserstein ball centered at the empirical distribution, thereby providing both finite sample guarantees and asymptotic consistency. My first model is a data-driven chance-constrained optimization in which a decision has to be feasible with high probability under every distribution within the ambiguity set. My second model is a data-driven two-stage optimization that accounts for the deterministic immediate cost as well as the worst-case expected future cost. Both models are classical in the literature of decision-making under uncertainty and are NP-hard even if there is no ambiguity about the true data-generating distribution. I provide an exact deterministic reformulation for the first model, and I propose a high-quality randomized approximation scheme for the second model. The encouraging in-sample and out-of-sample performances, as well as the scalability of both models are demonstrated in numerical experiments portfolio selection, transportation, and newsvendor problems.