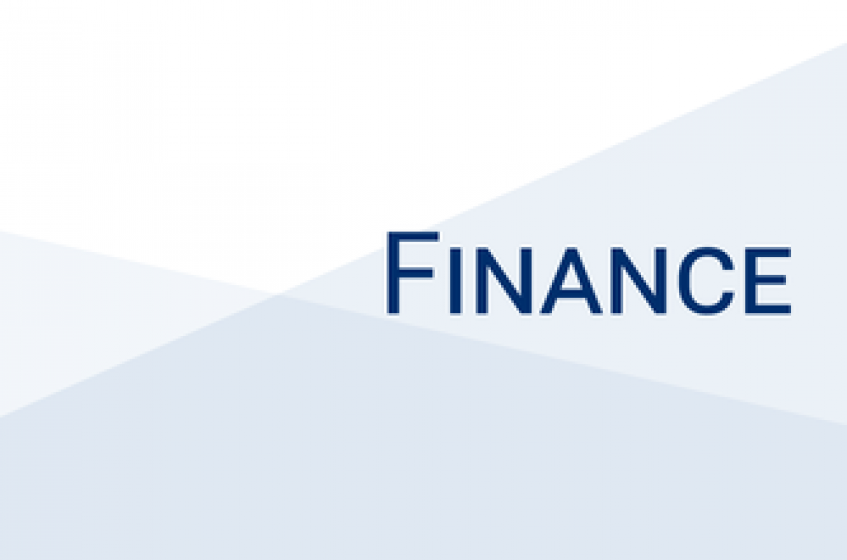
“Efficient Coding and Risky Choice” by Dr. Lawrence Jin
Assistant Professor of Finance
Division of the Humanities and Social Sciences
California Institute of Technology
We present a model of risky choice in which the decision maker (DM) perceives a lottery payoff with noise due to the brain’s limited capacity to represent information. We model perception using the principle of efficient coding, which implies that perception is most precise for frequently occurring stimuli. Our model shows that it is efficient for risk taking to be more sensitive to those payoffs that the DM encounters more frequently. The model also predicts that the DM’s value function fluctuates with the recently encountered distribution of payoffs. To test the model, we manipulate the distribution of payoffs in a laboratory experiment. We find that risk taking is indeed more sensitive to those payoffs that are presented more frequently. We then conduct an additional experiment to test efficient coding by incentivizing subjects to classify which of two symbolic numbers is larger. We find that subjects exhibit higher accuracy for those numbers that they have observed more frequently, providing further evidence that perception of a given numerical quantity varies with the recent environment. Overall, our experimental results suggest that risk taking depends systematically on the payoff distribution to which the DM’s perceptual system has recently adapted.