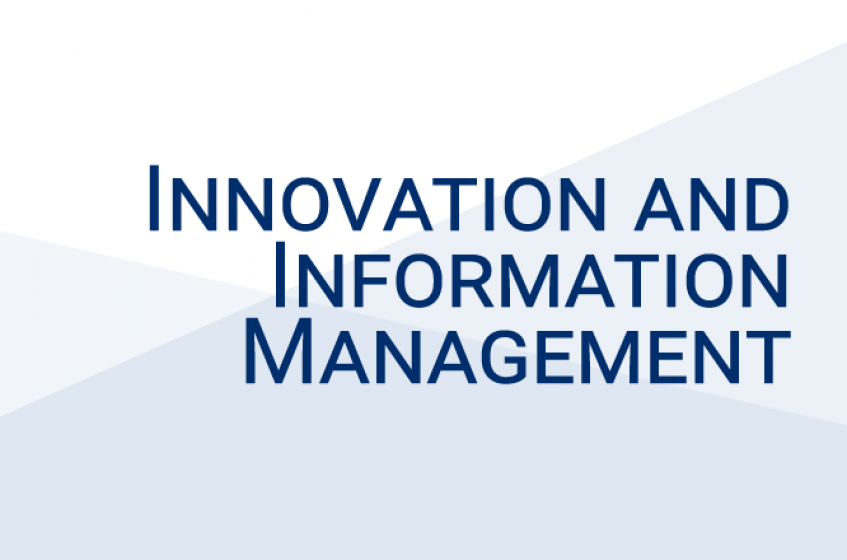
“Fake News Propagation and Detection: A Sequential Model” by Dr. Yiangos Papanastasiou
Dr. Yiangos Papanastasiou
Assistant Professor
Haas Operations and Information Technology Management Group
University of California Berkeley
In the wake of the 2016 US presidential election, social media platforms are facing increasing pressure to combat the propagation of "fake news" (i.e., articles whose content is fabricated). Motivated by recent attempts in this direction, we consider the problem faced by a social media platform that is observing the sharing actions of a sequence of rational agents and is dynamically choosing whether to conduct an inspection (i.e., a "fact-check") of an article whose validity is ex ante unknown. We first characterize the agents' inspection and sharing actions and establish that in the absence of any platform intervention, the agents' news-sharing process is prone to the proliferation of fabricated content, even when the agents are intent on sharing only truthful news. We then study the platform's inspection problem. We find that because the optimal policy is adapted to crowdsource inspection from the agents, it exhibits features that may appear a priori nonobvious; most notably, we show that the optimal inspection policy is nonmonotone in the ex ante probability that the article being shared is fake. We also investigate the effectiveness of the platform's policy in mitigating the detrimental impact of fake news on the agents' learning environment. We demonstrate that in environments characterized by a low (high) prevalence of fake news, the platform's policy is more effective when the rewards it collects from content sharing are low relative to the penalties it incurs from the sharing of fake news (when the rewards it collects from content sharing are high in absolute terms).