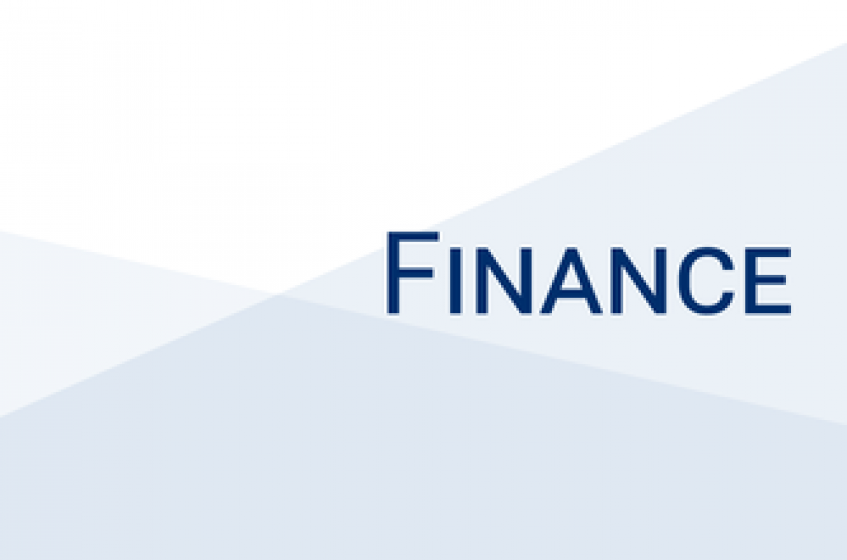
“Predictably Unequal? The Effects of Machine Learning on Credit Markets” by Dr. Paul Goldsmith-Pinkham
Assistant Professor
Yale School of Management
Yale University
Innovations in statistical technology, including in predicting creditworthiness, have sparked concerns about differential impacts across categories such as race. Theoretically, distributional consequences from better statistical technology can come from greater flexibility to uncover structural relationships, or from triangulation of otherwise excluded characteristics. Using data on US mortgages, we predict default using traditional and machine learning models. We find that Black and Hispanic borrowers are disproportionately less likely to gain from the introduction of machine learning. In a simple equilibrium credit market model, machine learning increases disparity in rates between and within groups; these changes are primarily attributable to greater flexibility.