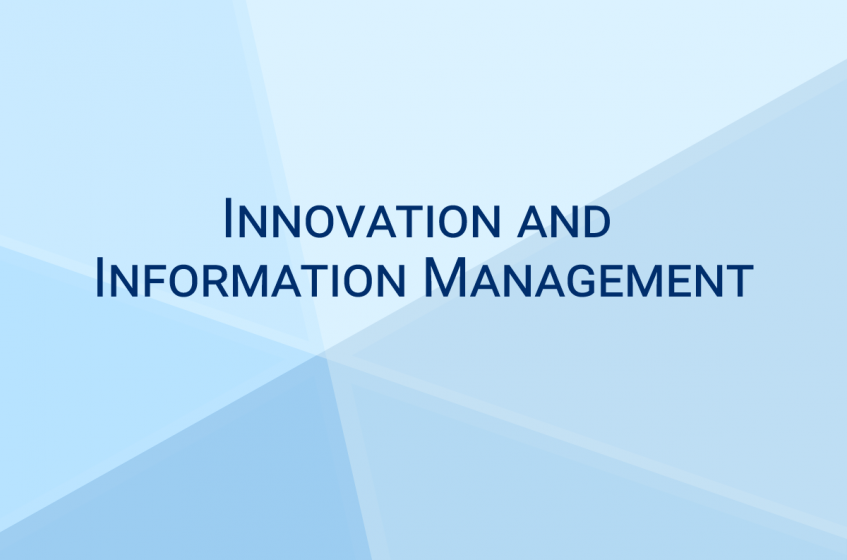
Scheduling with service-time Information: the power of two priority classes
With the development of statistical learning techniques, an important question to ask in operations management is how to utilize the customer-side information to achieve better system performance. In this work, we look into one particular such information – service times – and study how to use that information to do smarter scheduling for service systems. When having perfect service time information, the shortest remaining processing time first (SRPT) achieves superior performance. However, there are two main obstacles when implementing this policy in practice: (1) managing a queue where the sequence of customers may change upon each arrival is hard, and (2) we may not have perfect job-size information. Motivated by the main insights from SRPT, we propose an easy-to-implement two-class priority rule that achieves comparable performance to SRPT. When only predicted service times are available, we quantify how prediction errors affect the performance of the two-class priority rule. We show that even with very noisy service-time information, our policy still achieves substantial performance improvement over blind policies.