Problem definition: Providing fast and reliable delivery services is key to running a successful online retail business. To achieve a better delivery time guarantee policy, we study how to estimate and promise delivery time for new customer orders in real time. Academic/practical relevance: Delivery time promising is critical to managing customer expectations and improving customer satisfaction. Simply overpromising or underpromising is undesirable because of the negative impacts on short-/long-term sales. To the best of our knowledge, we are the first to develop a data-driven framework to predict the distribution of order delivery time and set promised delivery time to customers in a cost-effective way. Methodology: We apply and extend tree-based models to generate distributional forecasts by exploiting the complicated relationship between delivery time and relevant operational predictors. To account for the cost-sensitive decision-making problem structure, we develop a new split rule for quantile regression forests that incorporates an asymmetric loss function in split point selection. We further propose a cost-sensitive decision rule to decide the promised delivery day from the predicted distribution. Results: Our decision rule is proven to be optimal given certain cost structures. Tested on a real-world data set shared from JD.com, our proposed machine learning–based models deliver superior forecasting performance. In addition, we demonstrate that our framework has the potential to provide better promised delivery time in terms of sales, cost, and accuracy as compared with the conventional promised time set by JD.com. Specifically, our simulation results indicate that the proposed delivery time promise policy can improve the sales volume by 6.1% over the current policy. Managerial implications: Through a more accurate estimation of the delivery time distribution, online retailers can strategically set the promised time to maximize customer satisfaction and boost sales. Our data-driven framework reveals the importance of modeling fulfillment operations in delivery time forecasting and integrating the decision-making problem structure with the forecasting model.
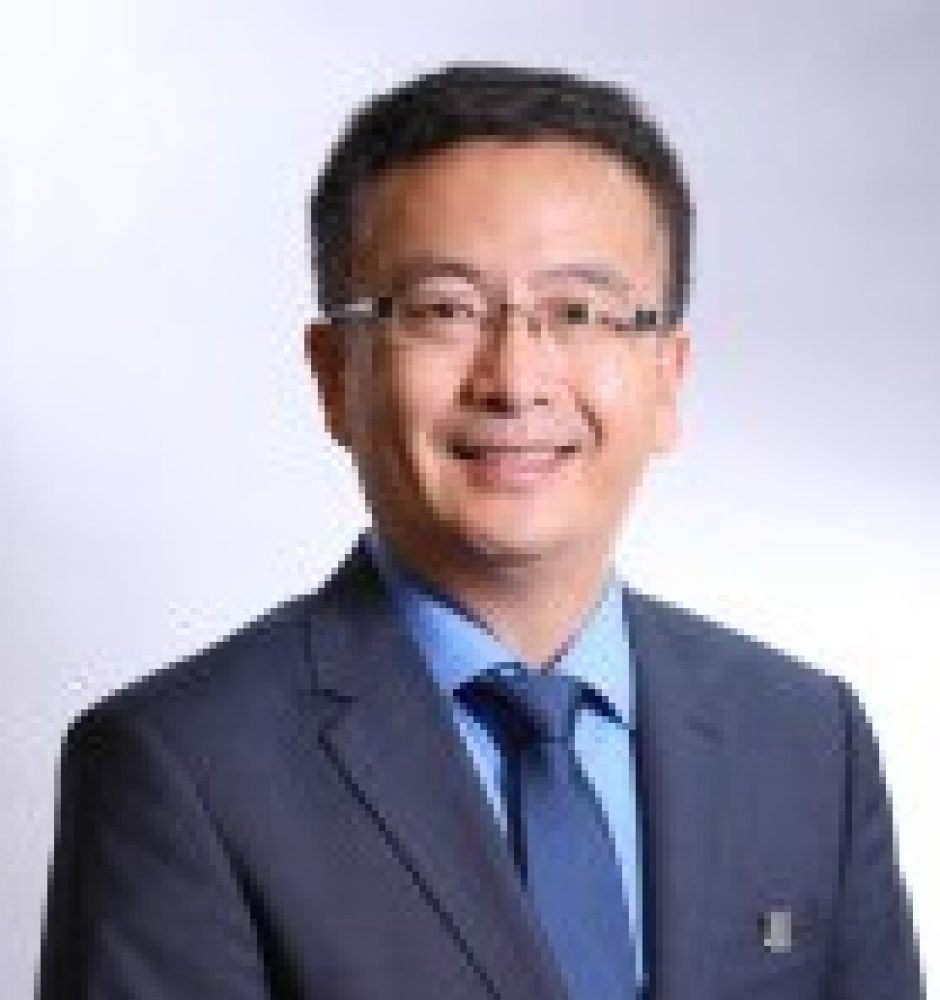
KB 1040
Professor Shen obtained his PhD from Northwestern University, USA in 2000. He started his academic career as Assistant Professor at the University of Florida in the same year, and joined the University of California, Berkeley in 2004, where he rose through the academic ranks to become Chancellor’s Professor and Chair of the Department of Industrial Engineering and Operations Research and Professor of the Department of Civil and Environmental Engineering. Professor Shen joined HKU in 2021. Internationally recognized as a top scholar in his field, Professor Shen is a Fellow of the Institute for Operations Research and the Management Sciences (INFORMS), a Fellow of the Production and Operations Management Society (POMS), a Fellow of the Hong Kong Academy of Engineering Sciences, and a former President of POMS.
- Integrated Supply Chain Design and Management
- Data Driven Logistics and Supply Chain Optimization
- Design and Analysis of Optimization Algorithms
- Energy Systems Optimization
- Transportation System Planning
- Associate editor for Operations Research
- Associate editor for Management Science
- Associate editor for Manufacturing & Service Operations Management
- Associate editor for Naval Research Logistics
- Associate editor for IIE Transactions
- Department editor for Production and Operations Management
- CAREER Award from NSF (2003)
- The Inaugural Chuck ReVelle Rising Star Award, SOLA, INFORMS (2008)
- IISE/Joint Publishers Book-of-the-Year Award (2013)
- Runner-up, INFORMS Health Application Society Best Paper Award (2016)
- Franz Edelman Laureate (2018)
- INFORMS Fellow (2018)
- M&SOM Best Paper Award (2019)
- POMS-JD.com Best Data-Driven Research Paper Award (2019)
- INFORMS TSL Best Paper Award (2019)
- Finalist, MSOM Data Driven Challenge (2020)
- Finalist, MSOM Data Driven Challenge (2021)
- Semifinalist, Gartner Power of the Profession Supply Chain Award (2022)
- POMS Fellow (2022)
- Fellow, Hong Kong Academy of Engineering Sciences (2022)
- Finalist, M&SOM Practice-based Research Competition (2023)
- Finalist, INFORMS Data Mining Best Paper Award (2023)
- Franz Edelman Laureate (2023)
- Gartner Power of the Profession Supply Chain Award (2024)
- Fundamentals of Supply Chain Theory, co-authored with Larry Snyder, was published by Wiley in August, 2011.
- Integrated Modeling for Location Analysis, co-authored with Ho-Yin Mak, Foundations and Trends in Technology, Information and Operations Management. Download the book
- Handbook of Supply Chain Analysis in the E-Business Era (Springer)
We study a risk-averse newsvendor problem where demand distribution is unknown. The focal product is new, and only the historical demand information of related products is available. The newsvendor aims to maximize its expected profit subject to a profit risk constraint. We develop a model with a value-at-risk constraint and propose a data-driven approximation to the theoretical risk-averse newsvendor model. Specifically, we use machine learning methods to weight the similarity between the new product and the previous ones based on covariates. The sample-dependent weights are then embedded to approximate the expected profit and the profit risk constraint. We show that the data-driven risk-averse newsvendor solution entails a closed-form quantile structure and can be efficiently computed. Finally, we prove that this data-driven solution is asymptotically optimal. Experiments based on real data and synthetic data demonstrate the effectiveness of our approach. We observe that under data-driven decision-making, the average realized profit may benefit from a stronger risk aversion, contrary to that in the theoretical risk-averse newsvendor model. In fact, even a risk-neutral newsvendor can benefit from incorporating a risk constraint under data-driven decision making. This situation is due to the value-at-risk constraint that effectively plays a regularizing role (via reducing the variance of order quantities) in mitigating issues of data-driven decision making, such as sampling error and model misspecification. However, the above-mentioned effects diminish with the increase in the size of the training data set, as the asymptotic optimality result implies.
Accessibility of Electric Vehicle (EV) charging stations is an important factor for adoption of EV, which is an effective green technology for reducing carbon emissions. Recognizing this, many governments are contemplating ideas for achieving EV adoption targets, such as constructing extra EV charging stations directly or offering subsidies to entice automakers to construct more EV charging stations. To achieve these targets, governments need to coordinate with automakers to ensure that the total number of charging stations is planned optimally. We study this coordination problem by considering the interactions among the government, automakers, and consumers, our equilibrium analysis yields three major results. First, both the government and the automaker should build extra EV charging stations when their construction costs are independent. Simultaneously, the government should offer a per-station subsidy to the automaker only when the adoption target and the construction cost are both high. However, when the construction costs are dependent, the government should delegate the construction to the automaker by offering a per-station subsidy. Second, when the government considers consumer purchase subsidy as an extra lever, we find that the purchase subsidy for consumers is more cost-effective than offering a per-station subsidy to the automaker. Third, the structure of the optimal government policy remains the same regardless of whether the government's goal is to improve EV adoption or consumer welfare. Our results can serve as guidelines for governments when contemplating coordination with automakers for the construction of EV charging stations to improve EV adoption as well as consumer welfare further.