在第七章中,周昭泷教授探讨了与人工智能相关的三大层面 — 香港的竞争力、劳动力市场和安全考量。 首先,要保持香港在全球的竞争力,各行各业都不能忽视人工智能的应用。面对其他亚太区城市的激烈竞争以及中美科技战,香港必须制定独特的策略,在基础研究和实际应用方面积累实力。其次,作者观察到这次的人工智能浪潮可能会对高技能、高收入工作造成影响。人工智能也将深远影响国际和本地的劳动力市场,在复杂的相互作用环境中,取代和创造林林总总的工作。第三,人工智能可能会对社会构成一定的安全问题,例如发生失误、遭受黑客攻击、病毒和软件程式错误、存在偏见及歧视等。因此,政府责无旁贷,应教育公众有关人工智能的力量和风险,并制定关于人工智能使用责任和伦理考量的规例和指引。
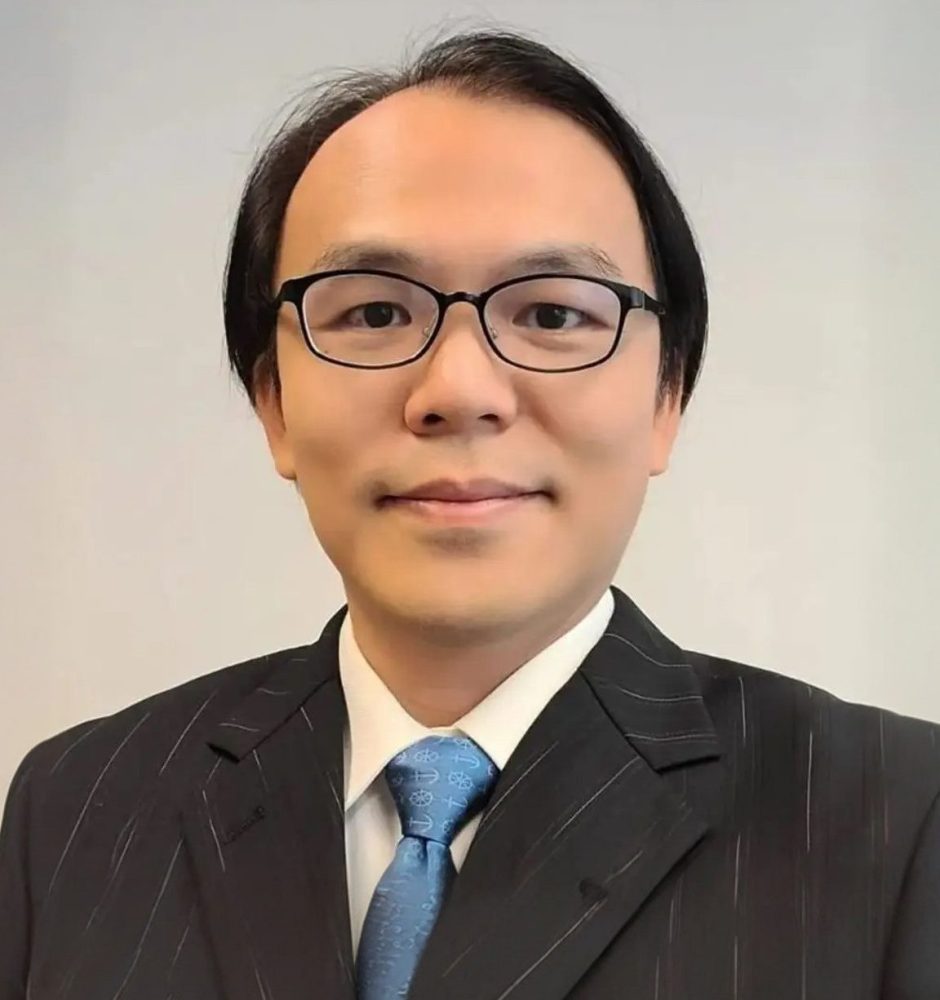
3917 1014
KK 1324
虽然先进的机器学习算法大大提升了金融欺诈检测研究的发展,然而,在构建有效辨识欺诈行为特征(或属性)方面仍然存在挑战。近年,在人们可以于没有金融中介的情况下向他人贷款的P2P 借贷平台上,出现了一种新型欺诈,因为信息不对称的问题在这些借贷市场更加严重。我们以舞弊三角论及其引伸的理论为基础,利用设计科学研究方法,除了参考借款人和贷款请求的基本特征以外,更直接以P2P 借贷交易数据构建了五类新的行为特征。这些行为特征,以研究欺诈者的贷款请求和还款记录、人际网络、投标过程的特征和活动次序为基础,并旨在评估其进行欺诈的能力、诚信和欺诈机会。我们使用涵盖中国两个大型 P2P 借贷平台的真实用户的数据集进行评估。研究结果显示,结合新的行为特征与基本特征可显著提升金融欺诈检测的表现。是项设计科学研究为金融欺诈检测的文献和实践带来了新见解。
情绪表达被网络新闻广泛地传播。现时在研究读者对网络新闻的看法时,主要聚焦语境线索对读者的推论和考量的影响;然而,愤怒、悲伤等分立情绪所扮演的角色却被忽视。 本文旨在填补相关研究领域的空隙,通过检视网络新闻所表达愤怒和悲伤的情绪,分析如何对读者造成影响。 利用情绪即社会信息(EASI)理论和评价倾向框架(ATF),我们发现网络新闻表达的愤怒情绪会降低其可信度。 但是,悲伤的情绪不会引起相同的效果。 研究另一个测试进一步发现,表达愤怒的情绪,可以解释为读者对作者认知心力的看法:读者认为,新闻标题表达愤怒的情绪,代表作者在撰写新闻时缺乏认知心力,令新闻可信度降低。 我们还展示了新闻可信度具有下游影响,可以影响各种社交媒体行为,包括阅读、喜欢、评论和分享。 本研究以网络新闻上的分立情绪(即愤怒、悲伤)为焦点,拓展了认知评估和人际影响层面的知识。 研究的结果具有实际意义,帮助社交媒体平台、新闻聚合平台和监管机构管理数字内容,防止假新闻传播。
Online auction markets host a large number of transactions every day. The transaction data in auction markets are useful for understanding the buyers and sellers in the market. Previous research has shown that sellers with different levels of reputation, as shown by the ratings and comments left in feedback systems, enjoy different levels of price premiums for their transactions. Feedback scores and feedback texts have been shown to correlate with buyers’ level of trust in a seller and the price premium that buyers are willing to pay (Ba and Pavlou 2002; Pavlou and Dimoka 2006). However, existing models do not consider the time-order effect, which means that feedback posted more recently may be considered more important than feedback posted less recently. This paper addresses this shortcoming by (1) testing the existence of the time-order effect, and (2) proposing a Bayesian updating model to represent buyers’ perceived reputation considering the time-order effect and assessing how well it can explain the variation in buyers’ trust and price premiums. In order to validate the time-order effect and evaluate the proposed model, we conducted a user experiment and collected real-life transaction data from the eBay online auction market. Our results confirm the existence of the time-order effect and the proposed model explains the variation in price premiums better than the benchmark models. The contribution of this research is threefold. First, we verify the time-order effect in the feedback mechanism on price premiums in online markets. Second, we propose a model that provides better explanatory power for price premiums in online auction markets than existing models by incorporating the time-order effect. Third, we provide further evidence for trust building via textual feedback in online auction markets. The study advances the understanding of the feedback mechanism in online auction markets.
Many people face problems of emotional distress. Early detection of high-risk individuals is the key to prevent suicidal behavior. There is increasing evidence that the Internet and social media provide clues of people’s emotional distress. In particular, some people leave messages showing emotional distress or even suicide notes on the Internet. Identifying emotionally distressed people and examining their posts on the Internet are important steps for health and social work professionals to provide assistance, but the process is very time-consuming and ineffective if conducted manually using standard search engines. Following the design science approach, we present the design of a system called KAREN, which identifies individuals who blog about their emotional distress in the Chinese language, using a combination of machine learning classification and rule-based classification with rules obtained from experts. A controlled experiment and a user study were conducted to evaluate system performance in searching and analyzing blogs written by people who might be emotionally distressed. The results show that the proposed system achieved better classification performance than the benchmark methods and that professionals perceived the system to be more useful and effective for identifying bloggers with emotional distress than benchmark approaches.