新冠肺炎疫情影响了不少人的生涯规划,但亦令人有幸重新审视自己的人生。王博士借此契机深思熟虑后,毅然放弃在金融界的事业,重投学者生涯,于2021年7月加入港大经管学院,担任创新及资讯管理学助理教授。
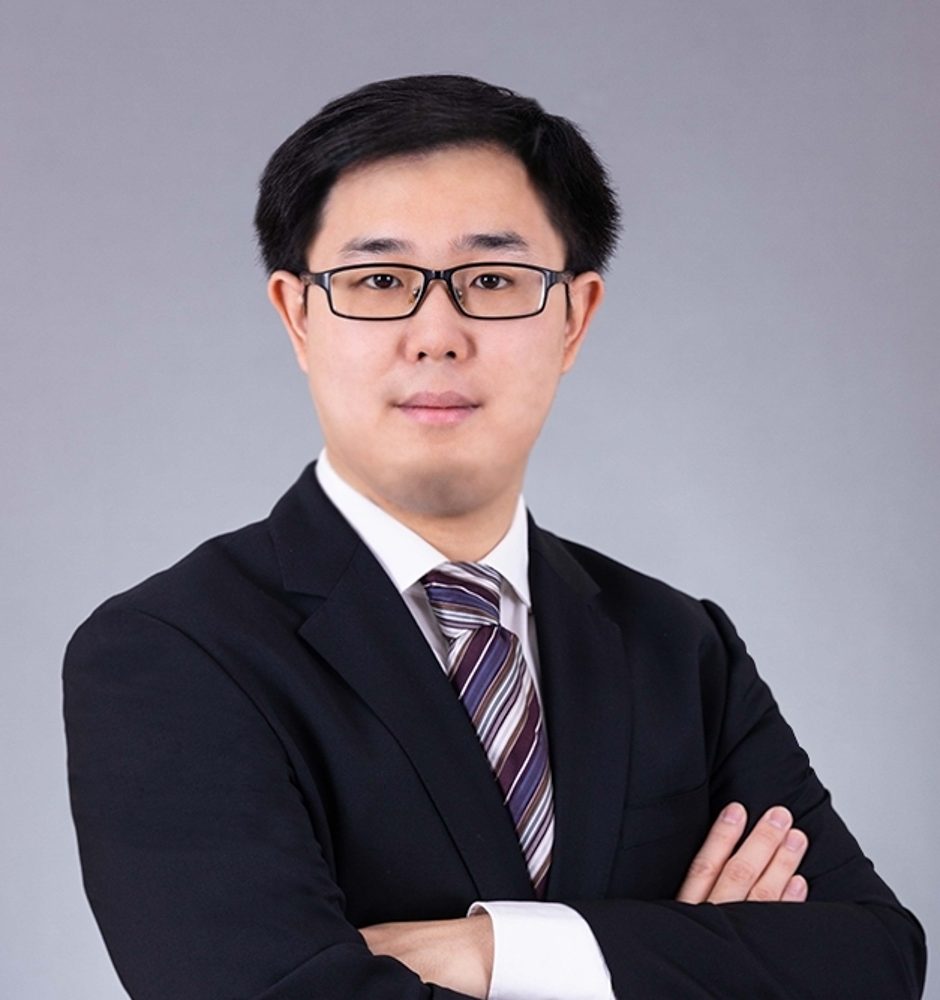
3917 1617
KK 1319
- PhD in Operations Research and Financial Engineering, Princeton University, 2016
- BS in Mathematics and Physics, Tsinghua University, 2011
Dr. Weichen Wang joined HKU in 2021 as an Assistant Professor. He obtained his PhD in Operations Research and Financial Engineering from Princeton University in 2016. After graduation, he joined Two Sigma Investments as a quantitative researcher where he worked on applying machine learning for equity market forecasting. Dr. Wang also served as a Visiting Lecturer at Princeton University for Spring 2020. Before his PhD, he received his bachelor’s degree in Mathematics and Physics from Tsinghua University in 2011.
Dr. Wang’s research areas include big data analysis, econometrics, statistics and machine learning, and he is particularly interested in the factor structure of the financial market and real-world applications of machine learning and deep learning. His works have been published in top journals including Annals of Statistics, Journal of Machine Learning Research, Journal of Econometrics etc.
- Business Statistics
- Research Methodologies in Business Analytics
- Quantitative Trading / AI in Finance
- Big data analysis
- Machine learning
- Econometrics and asset pricing
- Factor model and low-rank structure
- Semi-parametric and robust statistics
- Fan, J., Lou, Z., Wang, W., & Yu, M. (2025). Spectral Ranking Inferences Based on General Multiway Comparisons. Operations Research, forthcoming.
- Chen, X., Liao, Y., & Wang, W., (2024). Inference on Time Series Nonparametric Conditional Moment Restrictions Using Nonlinear Sieves. Journal of Econometrics, forthcoming.
- Fan, J., Lou, Z., Wang, W., & Yu, M. (2024). Ranking Inferences Based on the Top Choice of Multiway Comparisons. Journal of the American Statistical Association, forthcoming.
- Wang, W., An, R., & Zhu, Z. (2024). Volatility Prediction Comparison via Robust Volatility Proxies: An Empirical Deviation Perspective. Journal of Econometrics, 239(2), 105633.
- Wang, W., Han, J., Yang Z., & Wang Z. (2021). Global Convergence of Policy Gradient for Linear-Quadratic Mean-Field Control/Game in Continuous Time. International Conference on Machine Learning (ICML), 10772-10782.
- Fan, J., Wang, W., & Zhu, Z. (2021). A Shrinkage Principle for Heavy-Tailed Data: High-Dimensional Robust Low-Rank Matrix Recovery. Annals of Statistics, 49(3), 1239-1266.
- Fan, J., Wang, W., & Zhong, Y. (2018). An l∞ Eigenvector Perturbation Bound and Its Application to Robust Covariance Estimation. Journal of Machine Learning Research, 18(207), 1–42.
- Fan, J., Liu, H., & Wang, W. (2018). Large Covariance Estimation through Elliptical Factor Models. Annals of Statistics, 46(4), 1383–1414.
- Wang, W., & Fan, J. (2017).Asymptotics of Empirical Eigen-Structure for High Dimensional Spiked Covariance. Annals of Statistics, 45(3), 1342–1374.
- Fan, J., Liao, Y., & Wang, W. (2016). Projected Principal Component Analysis in Factor Models. Annals of Statistics, 44(1), 219–254.