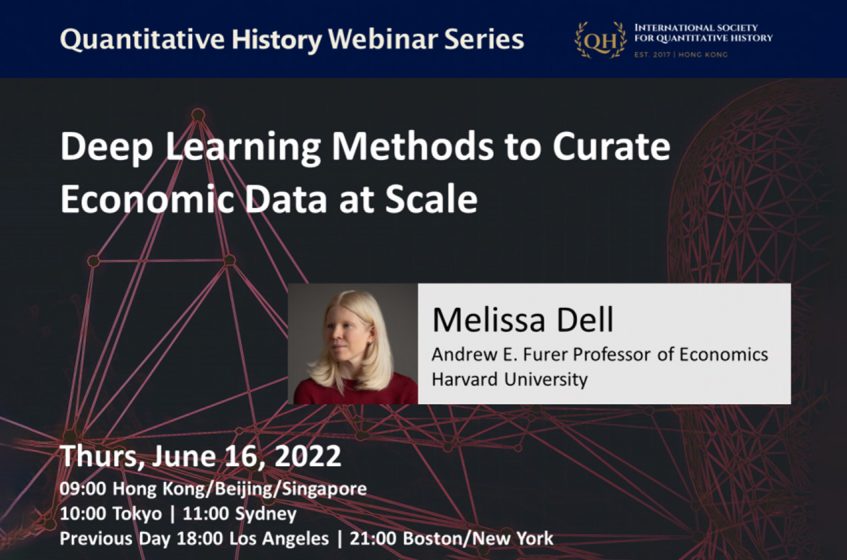
Deep Learning Methods to Curate Economic Data at Scale
Deep Learning Methods to Curate Economic Data at Scale
Vast amounts of data are trapped in non-computable formats, such as document image scans and text. Deep learning has the potential to greatly expand the questions that economists can study by providing rigorous methods for converting non-computable information into structured, computable data. Combined with advances in GPU compute and inexpensive cloud compute, this makes it feasible to process data on a massive scale.
In this Quantitative History Webinar, Melissa Dell of Harvard University will provide an overview of her recent work to develop deep learning methods and tools for creating computable social science data, with an aim of making structured digital data more representative of documentary history. This work emphasizes lower resource contexts – for which there are few incentives for commercial technology – and encompasses novel approaches and tools for document layout analysis, OCR, and NLP pipelines.
Live on Zoom on Thurs, June 16, 2022
09:00 Hong Kong/Beijing/Singapore | 10:00 Tokyo | 11:00 Sydney
Previous Day 18:00 Los Angeles | 21:00 Boston/New York (Wed, June 15)
Calculate your local time
The Quantitative History Webinar Series, convened by Professor Zhiwu Chen and Dr. Chicheng Ma of The University of Hong Kong (HKU), aims to provide researchers, teachers and students with an online intellectual platform to keep up to date with the latest research in the field, promoting the dissemination of research findings and interdisciplinary use of quantitative methods in historical research. The Series is co-organized by the International Society for Quantitative History, HKU Business School, and the Asia Global Institute (AGI).
Conveners:
Professor Zhiwu Chen
Dr. Chicheng Ma