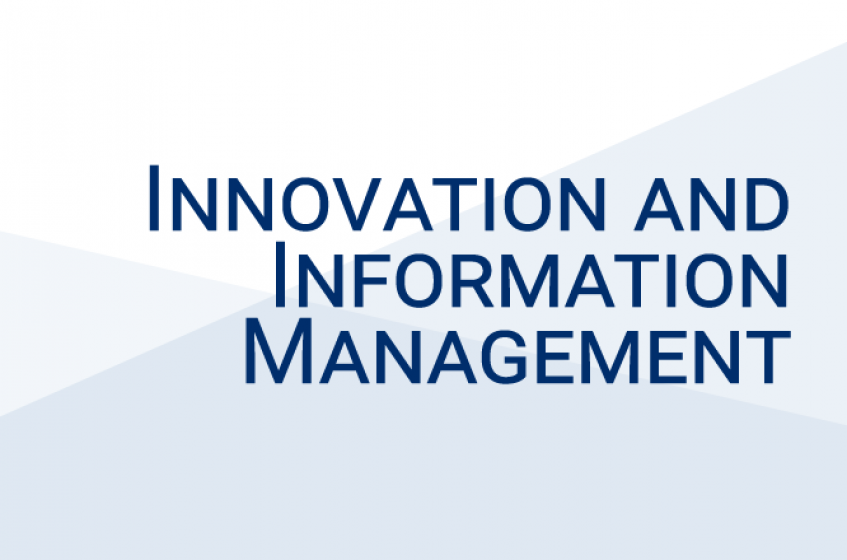
“Dynamic Pricing with Demand Learning: The Effect of Varying Cost” by Professor Jeff Hong
Professor Jeff Hong
Chair Professor of Management Sciences
College of Business
City University of Hong Kong
We study a dynamic pricing problem where the observed cost at each selling period varies from period to period while the demand function is unknown and independent of the observed cost. The decision maker needs to select a price from a menu of K prices in each period to maximize the expected cumulative profit. Motivated by the classical upper confidence bound (UCB) policy for multi-armed bandit problem, we propose a UCB-like policy to select the price. When the cost varies continuously, we show that the expected cumulative regret grows in the order of (logT)2, where T is the number of selling periods. When the cost takes discrete values in a finite set and all prices are optimal for some costs, we show that the expected cumulative regret is upper bounded by a constant for any T. For the case with continuous cost, we also develop a forced sampling (FS) policy whose expected cumulative regret grows in the order of log T. In spite of having a slower asymptotic growth rate, FS requires a problem-dependent parament as an input and its practical performance appears worse than the UCB-like policy for reasonably large T. Taking into account these factors, we suggest to use the UCB-like policy no matter whether the cost is continuous or discrete or unknown.