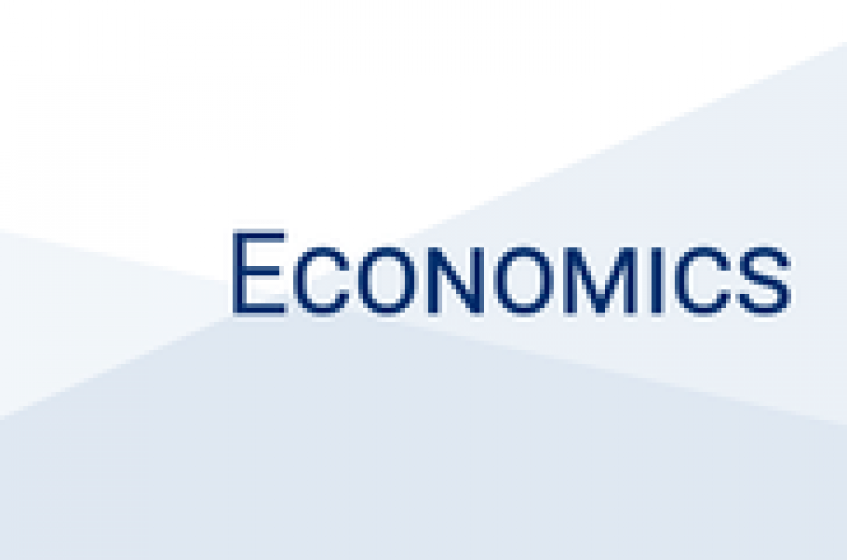
“Learning and Technology Growth Regimes” by Dr. Christian Matthes
Christian Matthes
Federal Reserve Bank of Richmond
Total factor productivity (TFP) and investment specifiic technology (IST) growth in the United States both show distinct regime-switching behavior, but while estimates of technology growth may be observable, the underlying regime is not and must be learned or inferred over time. In order to embed this learning process within a structural model, we develop a general perturbation-based solution method for dynamic stochastic general equilibrium models where agents within the model receive signals, but do not directly observe the regime. Our solution procedure includes a refinement to the partition perturbation method used to solve similar full-information regime-switching models, shows how to define a steady state in the environment, highlights an irrelevance result for first-order approximations to the solution, and produce higher-order approximations that have high accuracy. Then, using a real business cycle model with TFP and IST shocks that match our estimated regime-switching processes, we study the implications of learning about technology growth regimes. Learning lowers the volatility of output and investment growth while raising that of consumption growth; precautionary motives imply learning raises the level of output, and learning operates mainly by muting the response to regime switches.