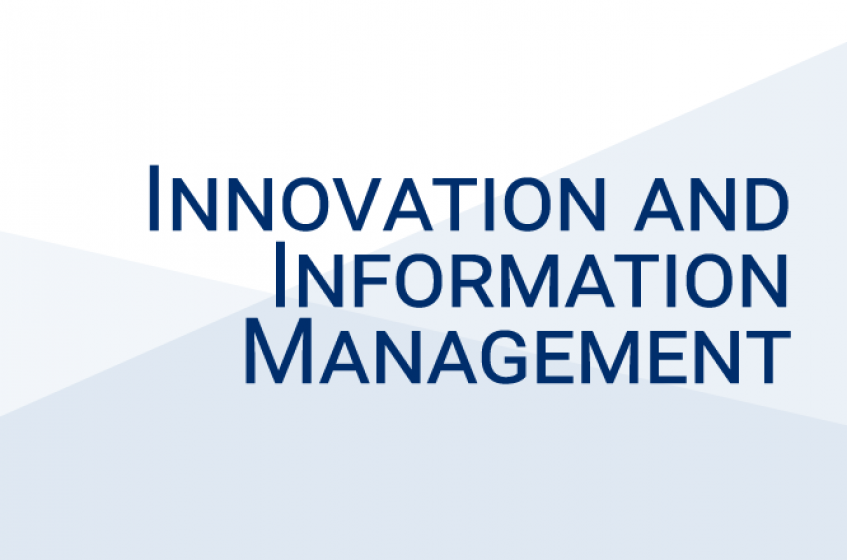
“Observational vs Experimental Data When Making Automatic Decisions Using Machine Learning” by Mr. Carlos Fernández
Ph.D. Candidate in Information Systems
Stern School of Business
New York University
With the recent explosion in both data and computing power, machine learning algorithms are increasingly used to make decisions automatically. These decisions are often causal in nature with the goal of improving an outcome by means of an intervention. Common examples include influencing someone’s purchasing behavior with an advertisement or increasing customer retention with a special offer. Unfortunately, if these algorithms use observational data to estimate the effect of the interventions, the resulting estimates will likely suffer from confounding bias. Investing in experimental data offers a way to estimate effects without confounding bias, but such data are costly and may be in short supply. This paper addresses the question of whether it would be better to invest in costly experimental data or use the readily-available (but confounded) observational data. We present a theoretical comparison between the use of observational and experimental data when the goal is to build models to make automated intervention decisions. The key insight of the work is that optimizing to make the correct decision generally involves understanding whether a causal effect is above or below a given threshold, which is different from optimizing to reduce the magnitude of the bias in a causal-effect estimate. As a result, models trained with confounded observational data may lead to decisions that are just as good (or better) in certain scenarios, such as when larger causal effects are more likely to be overestimated or when the benefits of larger and cheaper data outweigh the detrimental effect of confounding. The theoretical results are tested by comparing the two approaches using the wide variety of benchmark data sets (7,700 in total) from the 2016 ACIC causal modeling competition. Finally, we suggest that sensitivity analysis may be used in practice to determine whether collecting experimental data to improve treatment assignments would be cost-effective, illustrating with a simple procedure that shows a “Goldilocks effect”: in the illustration, the size of the experiment has to be just right for the investment to be worthwhile.