Problem definition: Providing fast and reliable delivery services is key to running a successful online retail business. To achieve a better delivery time guarantee policy, we study how to estimate and promise delivery time for new customer orders in real time. Academic/practical relevance: Delivery time promising is critical to managing customer expectations and improving customer satisfaction. Simply overpromising or underpromising is undesirable because of the negative impacts on short-/long-term sales. To the best of our knowledge, we are the first to develop a data-driven framework to predict the distribution of order delivery time and set promised delivery time to customers in a cost-effective way. Methodology: We apply and extend tree-based models to generate distributional forecasts by exploiting the complicated relationship between delivery time and relevant operational predictors. To account for the cost-sensitive decision-making problem structure, we develop a new split rule for quantile regression forests that incorporates an asymmetric loss function in split point selection. We further propose a cost-sensitive decision rule to decide the promised delivery day from the predicted distribution. Results: Our decision rule is proven to be optimal given certain cost structures. Tested on a real-world data set shared from JD.com, our proposed machine learning–based models deliver superior forecasting performance. In addition, we demonstrate that our framework has the potential to provide better promised delivery time in terms of sales, cost, and accuracy as compared with the conventional promised time set by JD.com. Specifically, our simulation results indicate that the proposed delivery time promise policy can improve the sales volume by 6.1% over the current policy. Managerial implications: Through a more accurate estimation of the delivery time distribution, online retailers can strategically set the promised time to maximize customer satisfaction and boost sales. Our data-driven framework reveals the importance of modeling fulfillment operations in delivery time forecasting and integrating the decision-making problem structure with the forecasting model.
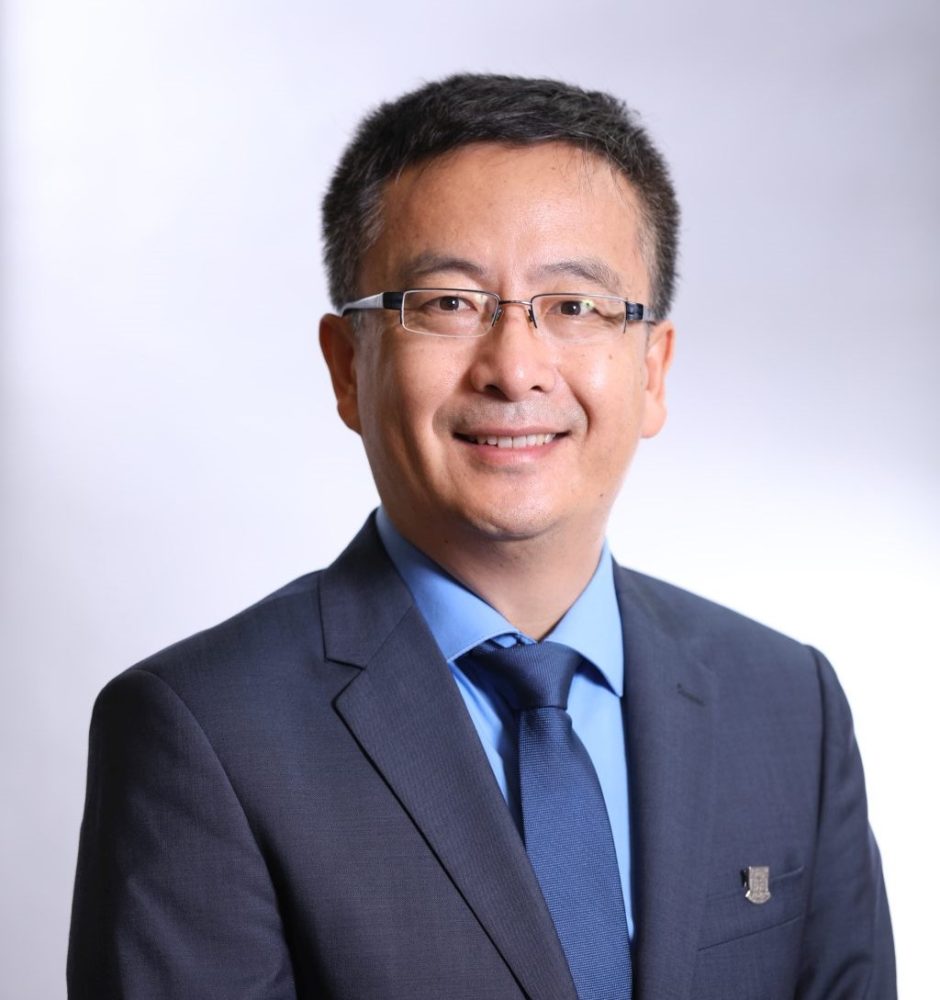
KB 1040
Professor Shen obtained his PhD from Northwestern University, USA in 2000. He started his academic career as Assistant Professor at the University of Florida in the same year, and joined the University of California, Berkeley in 2004, where he rose through the academic ranks to become Chancellor’s Professor and Chair of the Department of Industrial Engineering and Operations Research and Professor of the Department of Civil and Environmental Engineering. Professor Shen joined HKU in 2021. Internationally recognized as a top scholar in his field, Professor Shen is a Fellow of the Institute for Operations Research and the Management Sciences (INFORMS), a Fellow of the Production and Operations Management Society (POMS), a Fellow of the Hong Kong Academy of Engineering Sciences, and a former President of POMS.
- Integrated Supply Chain Design and Management
- Data Driven Logistics and Supply Chain Optimization
- Design and Analysis of Optimization Algorithms
- Energy Systems Optimization
- Transportation System Planning
- Fundamentals of Supply Chain Theory, co-authored with Larry Snyder, was published by Wiley in August, 2011.
- Integrated Modeling for Location Analysis, co-authored with Ho-Yin Mak, Foundations and Trends in Technology, Information and Operations Management. Download the book
- Handbook of Supply Chain Analysis in the E-Business Era (Springer)
We study a risk-averse newsvendor problem where demand distribution is unknown. The focal product is new, and only the historical demand information of related products is available. The newsvendor aims to maximize its expected profit subject to a profit risk constraint. We develop a model with a value-at-risk constraint and propose a data-driven approximation to the theoretical risk-averse newsvendor model. Specifically, we use machine learning methods to weight the similarity between the new product and the previous ones based on covariates. The sample-dependent weights are then embedded to approximate the expected profit and the profit risk constraint. We show that the data-driven risk-averse newsvendor solution entails a closed-form quantile structure and can be efficiently computed. Finally, we prove that this data-driven solution is asymptotically optimal. Experiments based on real data and synthetic data demonstrate the effectiveness of our approach. We observe that under data-driven decision-making, the average realized profit may benefit from a stronger risk aversion, contrary to that in the theoretical risk-averse newsvendor model. In fact, even a risk-neutral newsvendor can benefit from incorporating a risk constraint under data-driven decision making. This situation is due to the value-at-risk constraint that effectively plays a regularizing role (via reducing the variance of order quantities) in mitigating issues of data-driven decision making, such as sampling error and model misspecification. However, the above-mentioned effects diminish with the increase in the size of the training data set, as the asymptotic optimality result implies.
電動車充電站的地點是否便利,是電動車普及的一項重要因素而電動車的普及是一項有效減少碳排放量的綠色科技。有見及此,不少地方政府都想方設法,達到電動車普及化的目標,例如直資興建額外的電動車充電站或以資助方式吸引汽車製造商興建更多電動車充電站。為此,政府需與汽車製造商協調,以確保充電站的數量達到最佳的規劃。透過研究政府、汽車製造商及消費者的互動,我們總結三方互動過程中所產生的協調問題。平衡分析得出三個主要結論:第一,在各方獨立承擔建築成本的情況下,政府及汽車製造商均應興建額外的電動車充電站。而且,只有當興建電動車充電站的目標數量及建築成本都處於高水平時,政府才應向汽車製造商就興建每個充電站提供資助。然而,若汽車製造商的建築成本取決於政府興建電動車充電站的數量,那麼政府便應委派汽車製造商興建充電站,並就興建每個興建的充電站提供資助。第二,若政府向消費者購買電動車提供額外補助,研究顯示這額外補助措施較向汽車製造商就興建每個充電站提供資助更具成本效益。第三,不論政府的目標是改善電動車普及度或消費者福利,最理想的政策的結構是一樣的。此項研究結果可供政府與汽車製造商協調興建電動車充電站時作參考,以進一步改善電動車普及度和加強消費者福利。