A common problem in econometrics, statistics, and machine learning is to estimate and make inference on functions that satisfy shape restrictions. For example, distribution functions are nondecreasing and range between zero and one, height growth charts are nondecreasing in age, and production functions are nondecreasing and quasi-concave in input quantities. We propose a method to enforce these restrictions ex post on generic unconstrained point and interval estimates of the target function by applying functional operators. The interval estimates could be either frequentist confidence bands or Bayesian credible regions. If an operator has reshaping, invariance, order-preserving, and distance-reducing properties, the shape-enforced point estimates are closer to the target function than the original point estimates and the shape-enforced interval estimates have greater coverage and shorter length than the original interval estimates. We show that these properties hold for six different operators that cover commonly used shape restrictions in practice: range, convexity, monotonicity, monotone convexity, quasi-convexity, and monotone quasi-convexity, with the latter two restrictions being of paramount importance. The main attractive property of the post-processing approach is that it works in conjunction with any generic initial point or interval estimate, obtained using any of parametric, semi-parametric or nonparametric learning methods, including recent methods that are able to exploit either smoothness, sparsity, or other forms of structured parsimony of target functions. The post-processed point and interval estimates automatically inherit and provably improve these properties in finite samples, while also enforcing qualitative shape restrictions brought by scientific reasoning. We illustrate the results with two empirical applications to the estimation of a height growth chart for infants in India and a production function for chemical firms in China.
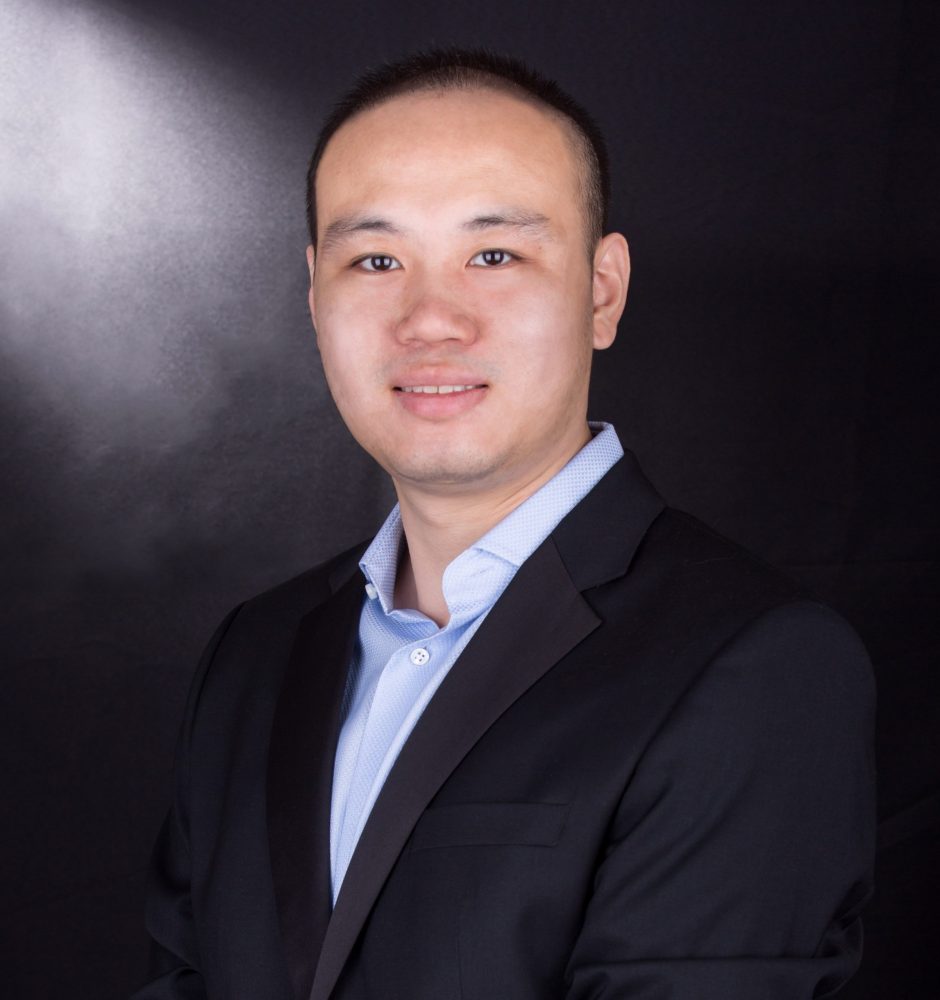
3917 0063
KK 826
Dr. Ye Luo received his Ph.D from the Massachusetts Institute of Technology in 2015. He received his B.S. degree from the Massachusetts Institute of Technology in 2010, majoring in Mathematics and Economics. Before joining FBE of HKU, he worked as an assistant professor at the economics department in the University of Florida. Dr. Ye Luo’s main research interests include high dimensional econometrics/statistics, machine learning and its empirical applications in economics and finance, for example, applying AI algorithms to develop smart, adaptive automated trading systems, applying big data methods/machine learning in default risk prediction, dynamic demand prediction, etc. He also has an interest and expertise in natural language processing.
Dr. Ye Luo has research papers published/forthcoming at Econometrica, Journal of the Royal Statistical Society: Series B, American Economic Review, P&P, etc. Beyond Dr. Ye Luo’s academic research, he has a strong interest in connecting research in data science to the industry. He has given/been invited to give lectures at DiDi, ShunFeng Express, Novartis, etc.
- “Estimation and Inference of Treatment Effects with L2-Boosting in High-Dimensional Settings”, 2023, Journal of Econometrics, 234(2), 714-731, with Jannis Kueck, Martin Spindler and Zigan Wang
- “Shape-Enforcing Operators for Generic Point and Interval Estimators of Functions”, 2021, Journal of Machine Learning Research, 22(220), 1-42, with Xi Chen, Victor Chernozhukov, Iván Fernández-Val and Scott Kostyshak
- “Errors in the Dependent Variable of Quantile Regression Models”, 2021, Econometrica, 89(2), 849-873, with Jerry Hausman, Haoyang Liu and Christopher Palmer
- “The Sorted Effects Method: Discovering Heterogeneous Effects Beyond Their Averages”, 2018, Econometrica, 86(6), 1911-1938, with Victor Chernozhukov and Ivan Fernandez-Val
- “An Imputation-regularized Optimization Algorithm for High Dimensional Missing Data Problems and Beyond”, 2018, 80(5), 899-926, Journal of the Royal Statistics Society Series B, with Faming Liang, Bochao Jia, Jingnan Xue and Qizhai Li
- “Core Determining Class and Inequality Selection”, 2017, American Economic Review, 107(5), 274-277, with Hai Wang
- “L2-Boosting for Economic Applications”, 2017, American Economic Review, 107(5), 270-273, with Martin Spindler