「與其為個人創造財富,不如為世界創造改變」是李哲鵬博士的格言。李博士一直致力透過學術研究推動資訊科技和機器學習的創新和進步。
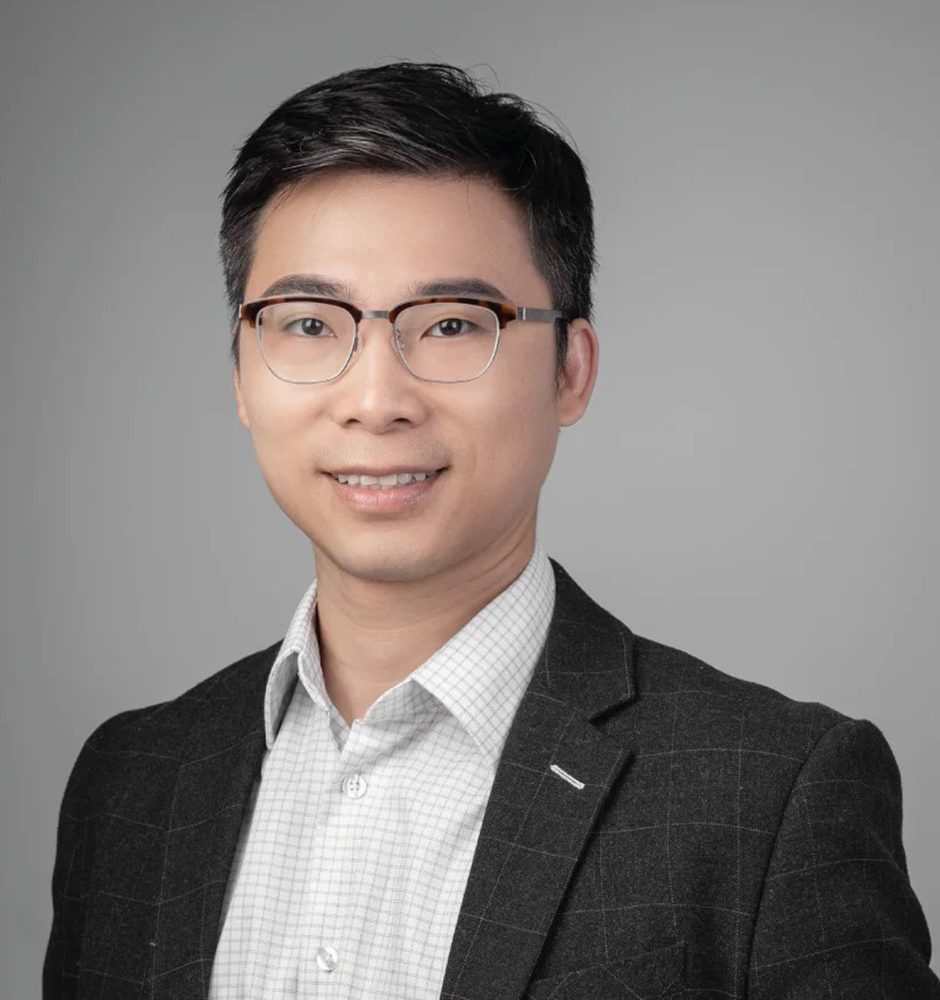
3910 2404
KK 822
- PhD in Operations & Information Systems, University of Utah, Salt Lake City, United States (Major in Information Systems, Minor in Computer Science)
- Master in Management Science & Engineering, University of Science and Technology of China, Hefei, China
Dr. Zhepeng (Lionel) Li is associate professor of Information Systems in the Faculty of Business and Economics. He received PhD degree in Operations and Information Systems from University of Utah in 2013. His research interests include computational data science, data mining, machine learning with applications in business analytics, recommendation and social network analytics. He has published in leading journals, such as Management Science, Information Systems Research, MIS Quarterly and ACM Transactions. His study is currently supported by the Discovery Grant of Natural Sciences and Engineering Research Council of Canada (NSERC) and National Natural Science Foundation of China (NSFC). He has received ISS design science research award of INFORMS in 2016 and served as organizer in the global research events such as program co-chair of INFORMS workshop on Data Science 2019 and Winter Conference of Business Analytics 2020, and chair of AI cluster of INFORMS 2021.
In particular, my research work is at the intersection of applied machine learning and business analytics/intelligence. Topics include recommendations, social network analytics, target marketing, PropTech, HR analytics, financial analytics, and distributed data mining with privacy protection. My interests revolve addressing relevant business and managerial problems from machine learning, predictive modelling and other computational data science perspectives. In this vein, my research paradigm encompasses formulating business domain questions into data-driven problems, analyzing the theoretical efficacy of the proposed methods, devising machine learning methods and/or algorithms, and using realistic data sets for benchmarking evaluations, ablation tests, experiments, or other empirical analyses.
- Computational Data Science
- Artificial Intelligence in Businesses
- Recommender Systems
- Information Privacy & Security
- Zhepeng (Lionel) Li, Yong Ge, Xue Bai. 2021. What Will be Popular Next? Predicting Hotspots in Two-mode Social Networks. MIS Quarterly* (MISQ)
- Wade Cook, Wanghong Li, Zhepeng (Lionel) Li, Joe Zhu. 2021. Efficiency Measurement for Hierarchical Situations. Journal of the Operational Research Society (JORS).
- Wade Cook, Wanghong Li, Zhepeng (Lionel) Li, Liang Liang, Joe Zhu. 2020. Efficiency Measurement with Products and Partially Desirably Co-Products. Journal of the Operational Research Society (JORS) 71(2): 335-345.
- Zhepeng (Lionel) Li, Xiao Fang, and Olivia R. Liu Sheng. 2018. A Survey of Link Recommendation for Social Networks: Methods, Theoretical Foundations, and Future Research Directions. ACM Transactions on Management Information Systems (TMIS) 9(1): 1-26.
- Zhepeng (Lionel) Li, Xiao Fang, Xue Bai, and Olivia R. Liu Sheng. 2017. Utility-based Link Recommendation for Online Social Networks. Management Science* (MS) 63(6): 1938-1952.
- Wanghong Li, Zhepeng (Lionel) Li, Liang Liang, Wade D. Cook. 2017. Evaluation of Ecological Systems and the Recycling of Undesirable Outputs: An Efficiency Study of Regions in China. Socio-Economic Planning Sciences 60: 77-86.
- Xiao Fang, Paul Hu, Zhepeng (Lionel) Li and Weiyu Tsai (Authors are of Equal Contribution). 2013. Predicting Adoption Probabilities in Social Networks. Information Systems Research* (ISR) 24(1): 128-145.
(* Area top-tier outlets)
- INFORMS ISS Design Science 2016
- PI, Discovery Grant, Natural Sciences and Engineering Research Council (NSERC) of Canada, 2017-2022
- Co-PI, National Natural Science Foundation (NSF) of China, 2017-2020
In social networks, social foci are physical or virtual entities around which social individuals organize joint activities, for example, places and products (physical form) or opinions and services (virtual form). Forecasting which social foci will diffuse to more social individuals is important for managerial functions such as marketing and public management operations. In terms of diffusive social adoptions, prior studies on user adoptive behavior in social networks have focused on single-item adoption in homogeneous networks. We advance this body of research by modeling scenarios with multi-item adoption and learning the relative propagation of social foci in concurrent social diffusions for online social networking platforms. In particular, we distinguish two types of social nodes in our two-mode social network model: social foci and social actors. Based on social network theories, we identify and operationalize factors that drive social adoption within the two-mode social network. We also capture the interdependencies between social actors and social foci using a bilateral recursive process—specifically, a mutual reinforcement process that converges to an analytical form. Thus, we develop a gradient learning method based on a mutual reinforcement process that targets the optimal parameter configuration for pairwise ranking of social diffusions. Further, we demonstrate analytical properties of the proposed method such as guaranteed convergence and the convergence rate. In the evaluation, we benchmark the proposed method against prevalent methods, and we demonstrate its superior performance using three real-world data sets that cover the adoption of both physical and virtual entities in online social networking platforms.